MPE CDT Student Cohort 2014
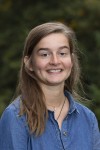
Lily Greig
Based at: University of Reading
Supervisors: David Ferreira (Reading)
Leads are fractures in sea ice. They provide a significant contribution to the polar heat balance despite making up only 5-10% of the sea ice cover, as gradients of sea ice concentration can result in lateral gradients in surface forcing and density gradients in the mixed layer. Through baroclinic instability, these fronts can energise submesoscale eddies. Submesoscale eddies have relatively fast time scales (hours to days), living in a parameter regime with finite Rossby and Richardson numbers. If energised they drive large horizontal exchange between ice-free and ice-covered ocean, and previous work showed that such dynamics could have an order 1 impact on the sea ice melt. Grid scales in the current generation of climate models are greater than the scale of submesoscale eddies and sea ice leads and ignore the effects of the sub-grid scale processes on the net polar heat balance. This project aims to explore these effects. It will start by building a mathematical model to develop understanding of the time and space scales of the density fronts formed under leads. Next it will explore under which conditions the density fronts may become unstable and spawn a submesoscale eddy field. Finally, this project will assess how subsmesoscale dynamics modulate air sea exchanges and if these processes should be included in climate models.
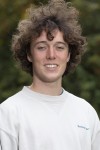
Calvin Nesbitt
Based at: University of Reading
Supervisors: Valerio Lucarini (Reading), Jeroen Wouters (Reading), Ted Shepherd (Reading)
The investigation of the response of GFD systems to perturbations is a key area of research within the mathematics of climate. Problems as different as the prediction of climate change, the understanding of the coupling between different climatic subsystems, the generation of low-frequency atmospheric variability from high-frequency weather noise, and the construction of parametrizations can be addressed using such a point of view. Multiscale systems feature specific mathematical challenges in the construction of response operators, as a result of the presence of slow decay of correlations for some variables, like the ocean in the case of climate.
Using techniques from ergodic theory and chaos, we will be looking at the response of a multiscale system of geophysical relevance to perturbations in the forcing. Moreover we intend to investigate from both from the point of view of deterministic and stochastic dynamics. For example we aim to use Ruelle Response theory to gain insight in the deterministic case. Additionally, we will look into the properties of multiscale systems. These are topics of great relevance in dynamical systems theory, GFD, and statistical mechanics.
We aim at using the modular MAOOAM climate quasi-geostrophic model, which allows for a great flexibility of configurations and is able to describe both atmospheric and oceanic dynamics in a simplified yet meaningful way. Understanding the geometry of the tangent space is key to the Ruelle formalism and we hope to understand this through the use of covariant Lyapunov vectors. This will allow us to project the response along the stable and unstable directions in the tangent space and so elucidate the applicability of the fluctuation-dissipation theorem. An example of a climatic problem we aim to investigate within this quasi-geostrophic framework would be the coupling between high-frequency, synoptic-scale weather noise and low-frequency, planetary-scale circulation variability. Note this is underpinned by a multiscale asymptotic theory.
This project has strong links with the ongoing debate on state dependent response in the context of climate research. Further areas for exploration would be the construction of unstable periodic orbits for the system and analysis of how they can be used to reconstruct the invariant measure of the system and its response. A third line of investigation deals with the study of the response of the correlations of the fields, rather than observables and the investigation of the nearing to the tipping points
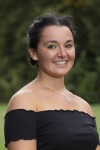
Chiara Cecilia Maiocchi
Based at: University of Reading
Supervisors: Valerio Lucarini (Reading), Andrey Gritsun (Russian Academy of Sciences)
Unstable periodic orbits (UPOs), have been proved to be a relevant mathematical tool in the study of Climate Science. In a recent paper Lucarini and Gritsun provided an alternative approach for understanding the properties of the atmosphere. Climate can be interpreted as a non-equilibrium steady state system and, as such, statistical mechanics can provide us with tools for its study.
UPOs decomposition plays a relevant role in the study of chaotic dynamical system. There is an intrinsic difficulty in sampling UPOs. Newton-like approaches have been proposed in the literature. The issue with these methods is that they are computationally expensive and do not guarantee convergence. During the PhD we would like to develop a new methodology to sample UPOs.
The idea is to populate with trajectories particular areas of interest of the attractor, reducing computational costs at a minimum. Ragone et al. developed a large deviation algorithm to sample rare events in a climate model. An ensemble simulation is performed, where the trajectories start from independent initial conditions that sample the invariant measure of the model. After a fixed time period the simulation is stopped and a score function is associated to each trajectory, depending on the dynamic up to that point. The trajectories that are going towards the region of interest, according to the score function, are copied, and the ones that perform badly are killed. At this stage, the surviving trajectories are slightly perturbed, so that they can evolve differently, and resampling is iterated another time. We believe we could propose a similar approach for sampling UPOs in a climate model. Positive results of UPOs search algorithms can be found in the literature. In particular, Gritsun and Lucarini first implemented this approach in a geophysical setting.
Once computed the UPOs, we would like to use them to reconstruct the invariant measure of the system and study the response of the system to perturbations. A well known result shows that it is possible to evaluate the expectation value of a reference function as an average over the various UPOs, where each one is weighted depending on its instability. The least unstable orbit will have the largest contribution, since it affects more heavily the dynamic of the system. Pollicott and Vytnova provided an alternative decomposition of the average of a reference function in power series, where the coefficient can be computed in terms of periodic points. This decomposition provides a computational more efficient means of approximation. We would like to investigate further this approach on the chosen climate model.
In a first phase of the project the student will familiarise with the mathematical background, working with a simpler model such as Lorenz '96. The main aim will be understanding and applying known methodologies for finding UPOs. Those techniques will then be extended to the more complex model MAOOAM, which has been found to exhibit chaotic behaviour for some values of the parameters. The challenge will be implementing a multiscale approach on this mode, as we have that ocean dynamics and atmosphere dynamics run on completely different time scale. In the last part of the project, the computed UPOs will be used to reconstruct the invariant measure of the system and study its response to external forcing. The innovation in the project is brought by the idea of finding a connection between rare events algorithms literature and UPOs. As far as we know this has never been done.
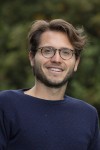
Niccolò Zagli
Based at: Imperial College London
Supervisors: Henrik Jensen (Imperial), G. Pavliotis (Imperial)
The aim of the project is to develop forecasting techniques in order to predict the onset of ecological crises. It is well known that these critical transitions (also known as tipping points) happen in many different areas, from ecological and natural systems to social and political ones. As a parameter is changed, for example the emission of CO2 or the global average temperature, the system becomes more and more unstable, meaning that a small fluctuation can lead it to a different, and usually more disastrous, state.
The project will proceed on two parallel paths. On one hand, since we still lack of a coherent mathematical framework describing these phenomena, we are going to borrow ideas from well-developed theories, such as statistical mechanics, the theory of phase transitions and the theory for fast/slow systems, to construct a solid toolbox to interpret critical transitions.
On the other hand, we are going to investigate methodologies that provide us a way to detect in time series data the onset of a crisis. Some work has already been done in this direction; however, robust data-driven indicators for these critical transitions are still missing. We are going to use the ideas developed in the more theoretical part of the project firstly on simulated data from models and then to real world data sets.
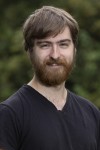
Ollie Street
Based at: Imperial College London
Supervisors: Dan Crisan (Imperial), Darryl Holm (Imperial), Matthew Piggott (Imperial)
The issue of ocean plastics has recently been much discussed by academics, policy makers, and environmental campaigners. The mathematical models which are used to describe the advection of plastics have largely ignored key factors such as sub-grid-scale dynamics and the mass of the debris. This raises the interesting question of how inertial particles move in a fluid governed by a SPDE. Using recent developments in stochastic fluid equations [Holm 2015] as a springboard, we will explore how the introduction of transport noise affects the properties (such as well posedness and smoothness) of a fluid model. In particular, can this type of noise restore uniqueness to a model? Furthermore, we will input the velocity field of the fluid into an equation which will return the velocity of the debris [Maxey & Riley, 1983], exploring the validity of doing this and whether this accurately models reality. Such a model would have applications in predicting the motion of ocean debris (such as icebergs, plastics, or aircraft wreckage) and, considering the model as an inverse problem, calibrating ocean models from drifter buoy data by understanding how the movement of the buoys differs from that of the fluid.
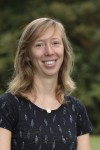
Swinda Falkena
Based at: University of Reading
Supervisors: Ted Shepherd (Reading), Jana de Wiljes (Potsdam), Antje Weisheimer (ECMWF)
Predictions of future climate change are usually represented as best estimates with an uncertainty range. However, at the regional scale, changes in atmospheric circulation play a large role and several outcomes may be possible. Under such conditions, an aggregate approach does not provide informative statements about risk. Storylines provide a way to represent the uncertainty in climate change itself, but need to be embedded within a probabilistic framework to account for the uncertainty in the particular realization of climate that will occur.
In this PhD project we use Bayesian causal networks to combine the storyline approach with probability. We focus on atmospheric circulation regimes in the Euro-Atlantic sector, since these have a large influence on the weather over Europe, and study their link with regional changes in extreme events. To inform the derivation of the causal network, expert knowledge will be used, which can be (partially) based on dynamical relationships derived from complex simulation models. The network will incorporate memory effects present in these dynamical relationships, which can give rise to persistent circulation anomalies. This will lead to a stronger physical foundation of the derived causal networks.
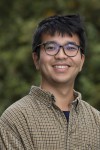
Ryo Kurashina
Based at: Imperial College London
Supervisor: Pavel Berloff (Imperial)
Geostrophic motions flowing over rough topography in the energetic Antarctic circumpolar current are known to be an important source of mixing, through both locally breaking lee waves and non-propagating vortical motions. This project will investigate the impact of these mechanisms on turbulent breakdown near rough bottom topography in order to better understand mixing characteristics in the Southern Ocean.
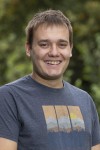
Oliver Phillips
Based at: University of Reading
Supervisors: Simon Chandler-Wilde (Reading), Chris Westbrook (Reading), Stephen Langdon (Brunel)
Linear wave scattering problems are ubiquitous in science and engineering applications. In the atmosphere, visible and ultraviolet radiation from the sun is scattered by ice crystals in cirrus clouds in the cold upper troposphere. These same clouds also scatter and absorb infrared radiation emitted from the earth's surface and lower troposphere. Together, these effects exert an important influence on the earth's radiation balance, and must be represented correctly in numerical climate models.
There are a number of unresolved problems with computing light scattering from an ice crystal in this regime. Firstly, the size of the ice particle is typically large compared to the wavelength of light illuminating it. This means that conventional numerical methods for such problems are prohibitively expensive. As a result the state-of-the-art in ice cloud radiative transfer is the use of ray-tracing (geometric optics). However, this approach cannot capture the effects of diffraction at the corners and edges of the ice crystal. This is typically overcome via a crude correction after computing the ray-tracing solution, but it is not clear whether this is accurate.
A second unresolved problem lies in the nature of the crystal surfaces themselves. Geometric optics is valid for surfaces which are flat and smooth. Real ice particles often have imperfections (roughness): such as steps, or pits, or in some cases may be rounded lumps. This roughness is in fact one of the leading order controls on the far field scattering pattern, and representing it properly is therefore a high priority. We would like to understand the influence of this roughness from a fundamental level, and therefore better constrain the way it is represented in radiative transfer.
The main tool for investigating these questions is the development of numerical methods for simulating light scattering that are able to capture the effects of diffraction whilst remaining computationally tractable across the frequency spectrum. One promising approach is the development of the class of methods known as "hybrid numerical-asymptotic boundary element methods" (HNABEM), which have been proven to be exceptionally efficient at solving a range of scattering problems, with various boundary conditions and geometries. To date though, the range of geometries for which these methods have been shown to be applicable is insufficient to address realistic questions in atmospheric science. This project aims to develop algorithms closely related to HNABEM yet containing key new ideas that will allow them to be applied to more general scattering problems, and to use these algorithms to investigate questions such as those posed above.
Firstly we will continue previous work on problems of scattering by multiple screens, with a goal being to consider scattering by a rough needle like ice crystals in two dimensions, and to compare qualitative results obtained with similar results for a standard three- dimensional solver so as to understand the potential usefulness of studying related two-dimensional problems. Secondly we will compare results obtained by state of the art approaches for high frequency three dimensional electromagnetic transmission problems in atmospheric physics (e.g., Physical Geometric-Optics Hybrid (PGOH) methods) with those obtained by standard numerical solvers, such as BEM++, so as to understand the significance of the diffraction missed by PGOH, and to develop ideas for approximating this difference via HNABEM.
The EPSRC research area that is of most relevance to the project is Numerical Analysis, with the project focused on the development, analysis and implementation of numerical methods for the solution of problems arising in atmospheric physics.
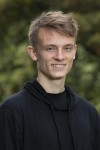
James Woodfield
Based at: University of Reading
Supervisors: Hilary Weller (Reading Meteorology), Colin Cotter (Mathematics, Imperial), Christian Kühnlein (ECMWF)
Transport, or advection, is arguably the most important part of an atmospheric prediction model. Everything in the atmosphere is transported by the wind - temperature, pollutants, moisture, clouds and even the wind itself (non-linear advection). Operational weather and climate centres, such as the Met Office and ECMWF, are developing new atmospheric dynamical cores to run on modern computer architectures and they need accurate, efficient and conservative advection schemes that are stable for long time steps suitable for their new models. Their current transport schemes are accurate and stable for long time steps but do not conservative. This project will develop implicit methods to achieve long stable time steps on arbitrary grids of the sphere for linear and non-linear problems. We will start by creating a model for Rayleigh-Benard convection and we will develop a Newton solver to achieve long, stable time steps.
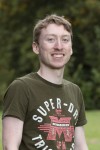
Sam Harrison
Based at: University of Reading
Supervisor: Alex Lukyanov (Reading)
Motivation
Deposition and dissolution geomorphic patterns are fundamental in the environment, and understanding the mechanisms underpinning their formation and evolution is central to paleo-reconstruction techniques used to probe past climatological systems. Such problems pose numerous mathematical challenges including motion of free boundaries (wall-shape as well as liquid-air interfaces), fluid-structure interactions and multiscale features in both space and time. The latter can pose particular challenges for lab experiments (for instance features can form over time scales of hundreds of years) and we propose a complete theoretical approach to quantify fundamental physical systems in order to compare with field observations.
Description, Mathematical Problems and Project Work Plan
We will begin with deposition problems and morphological instabilities, termed "crenulations", over calcite surfaces such as stalagmites, and also consider related dissolution problems. The formation mechanism is driven by a liquid film flowing down the structure and carrying slightly supersaturated calcium carbonate that deposits onto the wall. The change in wall-shape affects the flow and the flow affects the shape, albeit at different time scales. The Stokes/Navier-Stokes equations need to be addressed along with the underlying chemistry and surface growth models. We will use mathematical modelling, asymptotic analysis of partial differential equations, and numerical computations to advance the field in several crucial directions not considered previously.
In what follows we provide an overview of some of the mathematics along with an itemised work plan of the proposed thesis:
Realistic geometries: The state-of-the-art consists of modelling the stalagmite as a flat plate with an under-lying liquid film that naturally lead to Rayleigh-Taylor instabilities and absolute-convective transitions. The correct conical geometry is quite different from the flat plate picture.
New mathematical approaches: We will use full 3D axisymmetric geometries and analyse flows over slowly varying conical geometries using a combination of asymptotics and numerical simulations using finite-element and/or finite-volume methods. 3D axisymmetric geometries support waves that are not found in 2D and we propose to fully quantify the impact of such axial non-uniformities on the crenulation patterns. Our theoretical objectives are to analyse the flows in the appropriate geometries encountered in the field.
Novel instability mechanisms: The non-uniform 3D axisymmetric flows (that will be calculated during the project) over tapering conical geometries that are undergoing crenulation modulations are expected to support instabilities that have not been addressed previously. The spatiotemporal fluid structure interaction is expected to support new instabilities that will be analysed and compared with geomorphological features. Both linear and nonlinear analyses will be carried out.
Dissolution flows: A related parallel study of dissolution flows is also proposed. Here the flow sculpts the boundary at a rate that depends on the local fluid shear at the wall, leading to a dynamic fluid-structure interaction that is important in determining the global flow behaviour, for example laminar to turbulent transitions. Several mathematical issues remain to be studied, such as corner formation in dissolution flows past solid objects. In the vicinity of such features we propose to use matched asymptotic expansions in the spirit of triple-deck theory in order to provide the correct regularisation of the singularity and evaluate its effect on the global dynamics.
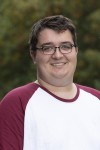
Tom Gregory
Based at: Imperial College London
Supervisors: Colin Cotter (Imperial), David Ham (Imperial)
Building an ocean model using compatible finite element numerics. This will begin with the following steps:
1. extend the linear incompressible nonhydrostatic Boussinesq implicit solver to include free surface (using the combined pressure-free surface approach.
2. Plug this solver into the Gusto modelling system and use existing Gusto advection solvers to construct a semi-implicit nonlinear equation solver loop using the linear Boussinesq solver as the implicit solver step.
At this stage we will have a full numerical scheme for the nonlinear nonhydrostatic incompressible Boussinesq equations and will be able to investigate performance and accuracy through standard test problems.
Going beyond that we could then work on a number of different topics establishing this system as a useable ocean modelling research tool: salinity and equation of state, turbulence closures e.g. Gent-McWilliams, work towards
wind driven baroclinic gyres, representation of bathymetry, parallel scalability, stability over large timesteps, etc. The supervisory team includes a researcher who leads Gusto code development (Shipton) and an external
collaborator who has been using Firedrake to develop a coastal ocean model.
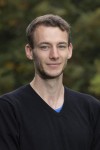
Philipp Breul
Based at: Imperial College London
Supervisors: Paulo Ceppi (Imperial), Pavel Berloff (Imperial)
A major challenge for climate science is to predict how atmospheric circulation will change as the planet warms. Current climate models generally predict a poleward shift of the midlatitude jet streams and storm tracks with warming. However, the climate science community faces two major difficulties in predicting future jet stream changes. First, the projected jet responses to greenhouse gas forcing are not robust in climate models; second, the dynamical mechanisms responsible for future jet shifts remain uncertain. Taken together, these two obstacles lead to particularly low confidence in climate model projections of jet stream changes.
The approach of my PhD project is to use a hierarchy of general circulation models to better understand the relationship between the initial state of the jet and its response to forcing. At the lowest order of this hierarchy we will use a barotropic model which represents the interaction of Rossby waves with the mean flow. The barotropic model findings will be used to interpret results from more realistic climate models. The model results will be interpreted physically using linear Rossby wave theory.
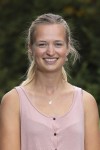
Lois Baker
Based at: Imperial College London
Supervisors: Ali Mashayek (Imperial), Graham Hughes (Imperial)
It is an emerging picture that deep ocean turbulence exerts a control over the climate system through regulating the oceanic uptake and redistribution of heat, carbon, nutrients and other tracers. Observations of such turbulence, and our ability to model it numerically, however, have been limited if non-existent until very recently. The challenge ahead is to understand physics of such turbulence to help represent them properly in climate models that are coarse resolution, hence incapable of resolving such processes.
The aim of this project is to better quantify the energy pathways from the mean geostrophic flow to dissipation and mixing through interactions with bottom topography, first through the generation of overturning lee waves above ridge-like topography and later through the generation of lee vortices at seamounts. Recent realistic and high resolution simulations of the Drake Passage provide an invaluable case study of flow topography interactions in an energetic and dynamically relevant area of the Southern Ocean. We will isolate these key processes from the simulations, analyse them for their contribution to energy pathways in the Southern Ocean, and create theoretical frameworks in order to enable better parametrisation of the processes in coarse resolution models.
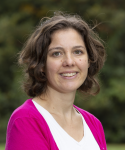
Cathie Wells
Based at: University of Reading
Supervisors: Paul Williams (Reading), Nancy Nichols (Reading), Ian Poll (Poll AeroSciences Ltd)
Air travel is the subject of much current controversy. Statistics for fuel use and CO2 emissions make uncomfortable reading for both airlines and environmental groups. Today’s flight routes avoid areas of strong headwinds and make use of available tailwinds, for a set optimal low fuel burn air speed. During the MRes phase of the project, however, it was shown that these trajectories do not always minimise fuel burn.
Airlines are keen to be able to provide a timetable that is unaffected by a particularly strong wind field. Delays are costly and early arrival can often result in extra fuel burn due to holding patterns. This PhD project will find optimal routes to minimise fuel burn for set departure and arrival times. Varying both airspeed and altitude, whilst considering the expected background wind field and the change in aircraft mass due to fuel burn, will provide a realistic model for the cruise phase of transatlantic flights.
Using Optimal Control theory, the dynamical system of routing equations derived in each situation can be solved numerically. The fuel burn statistics from the model can then be compared with recent actual flight data and recommendations made to the airline industry.
Lead supervisor: Paul Williams (Reading)
Co-supervisors: Dante Kalise (Imperial) and Nancy Nichols (Reading) Industrial co-supervisor: Ian Poll (Poll AeroSciences Ltd)
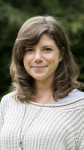
Elena Saggioro
Based at: University of Reading
Supervisors: Professor Ted Shepherd (Lead Supervisor, Department of Meteorology, University of Reading), Professor Sebastian Reich (Department of Mathematics and Statistics, University of Reading), Dr Jeff Knight (Met Office)
Project summary: Although there is confidence in thermodynamic aspects of global climate change for a given global warming, crucially there is still large uncertainty in the dynamical response at the regional scale. This is due to the role of atmospheric circulation, projected changes in which are poorly constrained by Global Climate models (GCMs) which give widely divergent responses, reflecting underlying model errors.
In order to identify the physical range of plausible responses, it is firstly necessary to identify models’ errors on short-timescale behaviour, for instance by comparing outputs with observed seasonal variability. Secondly, the connection between such errors and their spread in future projection needs to be understood and used to rule out unphysical projections. Within climate science this method is referred to as ‘emergent constraints’, its validity being rooted in the principles behind the fluctuationdissipation theorem (FDT) in statistical physics. Whilst promising, the application of emergent constraints in climate science has often failed, this due arguably to unsuitable practical estimations of both short-term errors and connection with long-term responses.
In this PhD we aim to tackle the issue of constraining the circulation response to climate change adopting time-series Bayesian Causal network (BCNs). This is a mathematical framework suitable to address questions related to causality, and its practical implementation results in a tool for robust statistical inference. A N-variate time evolving process can be associated to a time-series BCN by representing relations of pairwise conditional dependence in the process as lag-specific time-oriented links in the graph. The definition translates into a practical procedure for inferring causal links from data, once a test for conditional independence is chosen.
In the PhD we will use BCNs to estimate model errors on seasonal time scales, by comparing causal mechanisms as detected from reanalysis data with the same as extracted from model outputs. Then, we will connect short-timescale model errors to the long-term projection. The idea here is to complement the FDT-based reasoning with the insights in data provided by BCN representation.
Niraj Agarwal
Based at: Imperial College London
Principal Supervisor: Prof. Pavel Berloff (Department of Mathematics, Imperial College London)
Co-advisor: Peter Dueben, (ECMWF)
Summary: The oceanic turbulent circulation exhibits multiscale motions on very different space and time scales interacting with each other; e.g., jets, vortices, waves, and large-scale variability. In particular, mesoscale oceanic eddies populate nearly all parts of the ocean and need to be resolved in order to represent their effects on the general ocean and atmosphere circulations. However, capturing effects of these small-scale flows is highly challenging and requires non-trivial approaches and skills, especially when it comes to representing their effects in non-eddy resolving ocean circulation models. Therefore, the main goal of my project is to develop data-driven eddy parameterizations for use in both eddy-permitting and non-eddy-resolving ocean models. Dynamical models of reduced complexity will be developed to emulate the spatio-temporal variability of mesoscale eddies as well as their feedbacks across a large range of scales. These can serve as a low-cost oceanic component for climate models; and therefore the final aim of this project is to use the existing observational data to feed eddy parameterizations in comprehensive ocean circulation and climate models such as the ones used in global weather forecasts or in Climate Model Intercomparison Project(CMIP) models like CMIP7.
We will employ a variety of both common and novel techniques and methods of statistical data analysis and numerical linear algebra to extract the key properties and characteristics of the space-time correlated eddy field. The key steps involved in this framework are, a) first, find the relevant data-adaptive basis functions, i.e. the decomposition of time evolving datasets into their leading spatio-temporal modes using, for example, variance-based methods such as Principal Component Analysis (PCA) and, b) once the subspace spanned by above basis functions are obtained, we derive the evolution equations that emulate the spatio-temporal correlations of the system using methods such as nonlinear autoregression, artificial neural network, Linear Inverse Modelling (LIM), etc.
The proposed new science will help develop a state-of-the-art data-adaptive modelling framework for evaluation and application of Machine Learning and rigorous mathematical theory for dynamical and empirical reduction within the hierarchy of existing oceanic models.
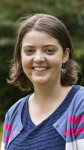
Mariana Clare
Based at: Imperial College London
Supervisors: Matthew Piggott (Lead supervisor, Department of Earth Science & Engineering, Imperial College London) and Colin Cotter (Department of Mathematics, Imperial College London). Industry supervisor: Dr Catherine Villaret (East Point Geo Consulting).
Summary: An estimated 250 million people live in regions that are less than 5 metres above sea level. Hence with sea level rise and an increase in both the frequency and severity of storms as a result of climate change, the coastal zone is becoming an ever more critical location for the application of advanced mathematical techniques. Models are currently used to assist in the design of coastal zone engineering projects including flood defences and marine renewable energy arrays. There are many challenges surrounding the development and application of appropriate coupled numerical models because they include both hydrodynamic and sedimentary processes and need to resolve spatial scales ranging from sub-metre to 100s of kilometres.
My project aims to develop and use advanced numerical modelling and statistical tools to improve the understanding of hazards and the quantification and minimisation of erosion and flood risk. Throughout this project, I will consider the hazards in the context of idealised as well as real world scenarios.
The main model I will use in my project is XBeach, which uses simple numerical techniques to compute dune erosion, scour around buildings and overwash. XBeach is also currently used, to a limited degree, with Monte Carlo techniques to generate a large number of storm events with different wave climate parameters. Uncertain atmospheric forcing is very important in erosion/scour processes and flood risk, which are intimately linked in many situations and cannot be considered in isolation. In my project I will explore how the new technique of Multi-level Monte Carlo simulations can be combined with XBeach to quantify erosion/flood risk. I am not only interested in the effects of extreme events, but also the cumulative effect of minor storm events for which Monte Carlo techniques are particularly appropriate. I will also explore how an adaptive mesh approach can be coupled with the statistical approach to assess the risk to coastal areas.
My project aims to develop and use advanced numerical modelling and statistical tools to improve the understanding of hazards and the quantification and minimisation of erosion and flood risk. Throughout this project, I will consider the hazards in the context of idealised as well as real world scenarios. The main model I will use in my project is XBeach, which uses simple numerical techniques to compute dune erosion, scour around buildings and overwash. XBeach is also currently used, to a limited degree, with Monte Carlo techniques to generate a large number of storm events with different wave climate parameters. Uncertain atmospheric forcing is very important in erosion/scour processes and flood risk, which are intimately linked in many situations and cannot be considered in isolation. In my project I will explore how the new technique of Multi-level Monte Carlo simulations can be combined with XBeach to quantify erosion/flood risk. I am not only interested in the effects of extreme events, but also the cumulative effect of minor storm events for which Monte Carlo techniques are particularly appropriate. I will also explore how an adaptive mesh approach can be coupled with the statistical approach to assess the risk to coastal areas.
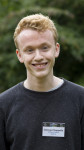
George Chappelle
Based at: Imperial College London
Supervisors: Martin Rasmussen (Imperial College London, Department of Mathematics), Jochen Broeker (University of Reading, Department of Mathematics and Statistics), Pavel Berloff (Imperial College London, Department of Mathematics)
Summary: The concept of a tipping point (or critical transition) describes a phenomena where the behaviour of a physical system changes drastically, and often irreversibly, compared to a small change in its external environment. Relevant examples in climate science are the possible collapse of the Atlantic Meridional Overturning Circulation (AMOC) due to increasing freshwater input, or the sudden release of carbon in peatlands due to an external temperature increase. The aim of this project is to develop the mathematical framework for tipping points and therefore contribute to a deeper understanding of them.
A number of generic mechanisms have been identified which can cause a system to tip. One such mechanism is rate-induced tipping, where the transition is caused by a parameter changing too quickly - rather than it moving past some critical value. The traditional mathematical bifurcation theory fails to address this phenomena. The goal of this project is to use and develop the theory of non-autonomous and random dynamical systems to understand rate-induced tipping in the presence of noise. A question of particular practical importance is whether it is possible to develop meaningful early-warning indicators for rate-induced tipping using observation data. We will investigate this question from a theoretical viewpoint and apply it to more realistic models.
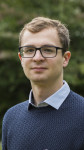
Stuart Patching
Based at: Imperial College London
Supervisors: Xue-Mei Li (Department of Mathematics, Imperial College London, Lead supervisor), Darryl Holm ( Department of Mathematics, Imperial College London), Dan Crisan (Department of Mathematics, Imperial College London)
Summary: The Gulf Stream can be thought of as a giant meandering ribbon-like river in the ocean which originates in the Caribbean basin and carries warm water across the Atlantic to the west coast of Europe, keeping the European climate relatively mild. In spite of its significance to weather and climate, the Gulf Stream has remained poorly understood by oceanographers and fluid dynamicists for the past seventy years. This is largely due to the fact that the large-scale flow is significantly affected by multi-scale fluctuations known as mesoscale eddies. It is hypothesised that the mesoscale eddies produce a backscatter effect which is largely responsible for maintaining the eastward jet extensions of the Gulf Stream and other western boundary currents.
The difficulty in modelling such currents lies in the high computational cost associated with running oceanic simulations with sufficient resolution to include the eddy effects. Therefore approaches to this problem have been proposed which involve introducing some form of parameterisation into the numerical model, such that the small scale eddy effects are taken into account in coarse grid simulations.
There are three main approaches we may consider in including this parameterisation: the first is stochastic advection, the second is deterministic roughening and the third is data-driven emulation.
These approaches have all be explored for relatively simple quasi-geostrophic ocean models, but we shall attempt to apply them to more comprehensive primitive equation models which have greater practical applications in oceanography. In particular we shall be using the MITgcm and FESOM2 models, to which we shall apply our parameterisations and run on a low-resolution grid and compare the results with high-resolution simulations.
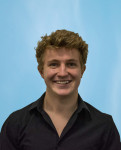
Louis Sharrock
Based at: Imperial College London
Supervisors: Nikolas Kantas (Department of Mathematics, Imperial College London, Lead supervisor), Professor Alistair Forbes (NPL)
Summary: This project aims to develop new methodology for performing statistical inference in environmental modelling applications. These applications require the use of a large number of sensors that collect data frequently and are distributed over a large region in space. This motivates the use of a space time varying stochastic dynamical model, defined in continuous time via a (linear or non-linear) stochastic partial differential equation, to model quantities such as air quality, pollution level, and temperature. We are naturally interested in fitting this model to real data and, in addition, on improving on the statistical inference using a carefully chosen frequency for collecting observations, an optimal sensor placement, and an automatic calibration of sensor biases. From a statistical perspective, these problems can be formulated using a Bayesian framework that combines posterior inference with optimal design.
Performing Bayesian inference or optimal design for the chosen statistical model may be intractable, in which case the use of simulation based numerical methods will be necessary. We aim to consider computational methods that are principled but intensive, and given the additional challenges relating to the high dimensionality of the data and the model, must pay close attention to the statistical model at hand when designing algorithms to be used in practice. In particular, popular methods such as (Recursive) Maximum Likelihood, Markov Chain Monte Carlo, and Sequential Monte Carlo, will need to be carefully adapted and extended for this purpose.
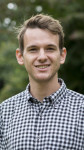
Adriaan Hilbers
Based at: Imperial College London
Supervisors: Prof Axel Gandy (Statistics Section, Department of Mathematics, Imperial College London), Dr David Brayshaw (Department of Meteorology, University of Reading)
In the face of climate change, considerable efforts are being undertaken to reduce carbon emissions. One of the most promising pathways to sustainability is decarbonising electricity generation and electrifying other sources of emissions such as transport and heating. This requires a near-total decarbonisation of power systems in the next few decades.
Making strategical decisions regarding future power system design (e.g. what power plant to build) is challenging for a number of reasons. The first is their complexity: electricity grids can be immensely complicated, making the effect of e.g. an additional power plant difficult to estimate. The second is the considerable uncertainty about future technologies, fuel prices and grid improvements. Finally, especially as more weather-dependent renewables are added, there is climate-based uncertainty: we simply don’t know what the future weather will be, or how well times of high demand will line up with times of high renewable output.
This project aims to both understand the effect of climate-based uncertainty on power system planning problems and develop methodologies for robust decision-making under these unknowns. This will be done in the language of statistics, using techniques such as uncertainty quantification, data reduction and decision-making under uncertainty. Furthermore, this investigation will employ power system models, computer programs simulating the operation of an electricity grid.
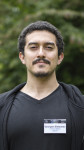
Georgios Sialounas
Based at: University of Reading
Supervisors: Tristan Pryer (University of Reading, Department of Mathematics and Statistics, Lead supervisor)
Summary: Hierarchical modelling is a common feature in many application areas. Indeed, most large scale geophysical simulations are built upon the basis of modelling phenomena with systems of PDEs. Depending on the application and the scale of the features needing to be simulated various levels of approximation are conducted, based on some underlying physical reasoning, resulting in a hierarchy of PDE models. At the top level of this hierarchy sits a PDE system that contains all information currently known about the process. For example, climate models contain a huge amount of information, including atmospheric composition, hydrology, impacts of ice sheets, human influence, vegetation, oceanographic aspects, solar inputs and so on. These extremely complicated mathematical models are far too complex to construct any analytical solution method for the resultant system, so, practically, reductions are made, with information being ignored so that the system has a lower complexity. Naturally, this reduction gives rise to hierarchies of models. I study how to make use of these hierarchies from the numerical perspective.
Alexander Alecio
Based at: Imperial College London
When modelling complicated physical systems such as the ocean/atmosphere system with relatively simple mathematical models based on (ordinary/partial, deterministic/stochastic) dierential equations, we expect some discrepancy between the mathematical model and the actual physical system. It is by now well understood that model error, plays an important role in the delity of the mathematical model and on its predictive capabilities. Model uncertainty, together with additional sources of randomness due, e.g. to incomplete knowledge of the current state of the system, sensitive dependence on initial conditions, parameterization of the small scales etc, should be taken into account when making predictions about the system under investigation.
In addition, many climatological models exhibit 'tipping points' - critical transitions where the output of the model changes disproportionately compared to the change in a parameter. [LHK+08] documents several, the most pertinent to British weather being the Stommel-Cessi box model for Atlantic thermohaline circulation, which suggests the collapse of the Atlantic Meridional Overturning Circulation, upon small changes in freshwater input.
Weather forecasting bodies overcome these inherent difficulties ensemble techniques (or probabilistic forecasting), running multiple simulations accounting for the range of possible scenarios. A forecast should then skilfully indicate the confidence the forecaster can have in their prediction, by accurately representing uncertainty [AMP13]. Clearly, model uncertainty can have a dramatic effect on the predictive capabilities of our mathematical model when we are close to a noise induced transition, a tipping point or a phase transition. This poses an important mathematical question: how can we systematically quantify the propogation of uncertainty through the model, from model parameters and initial conditions, to model-output, even in cases of 'tipping'?
[LHK+08] Timothy M. Lenton, Hermann Held, Elmar Kriegler, Jim W. Hall, Wolfgang Lucht, Stefan Rahmstorf, and Hans Joachim Schellnhuber. Tipping elements in the earth's climate system. Proceedings of the National Academy of Sciences, 105(6):1786{1793, 2008.
[AMP13] H. M. Arnold, I. M. Moroz, and T. N. Palmer. Stochastic parametrizations and model uncertainty in the Lorenz '96 system. Philosophical Transactions of the Royal Society of London Series A, 371:20110479{20110479, April 2013.
Supervisor: G.A. Pavliotis (Imperial College London); V. Lucarini (U. Reading)
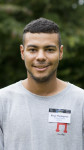
Rhys Leighton Thompson
Based at: University of Reading
Supervisors: Clare Watt, Department of Meteorology, Reading (Main), Paul Williams, Department of Meteorology, Reading (Co-Supervisor)
Abstract: Space Weather is the name given to the natural variability of the plasma and magnetic field conditions in near-Earth Space. 21st Century technology is increasingly reliant on space-based assets and infrastructure that are vulnerable to extreme space weather events. Due to the sparse nature of in-situ measurements, and the relative infancy of numerical space plasma physics models, we lack the ability to predict the timing and severity of space weather disruptions to either mitigate their effects, or adequately plan for their consequences.
In this project, we focus on important improvements to the numerical modelling of the Earth’s Outer Radiation Belt; a highly-variable region of energetic electrons in near-Earth space. In the Outer Van Allen Radiation Belt, electrons are trapped by the Earth's magnetic field and can be accelerated to a significant fraction of the speed of light. At such high energies, they pose significant hazards to spacecraft hardware. Most importantly for mankind’s reliance on space-based systems, the Outer Radiation Belt encompasses orbital paths that are of great use to society (e.g. geosynchronous orbit, and Medium Earth Orbits that contain global positioning and navigation systems).
The student will construct idealised numerical models of simple 1D diffusion problems with Dirichlet or Neumann boundary conditions and investigate their behaviour when appropriate stochastic parameterisations of diffusion coefficients are chosen. Initial and boundary values will be chosen to mimic realistic values in near-Earth space, and the solutions from the stochastic model will be compared with solutions from a traditional deterministic model. Given the novel nature of stochastic parameterisations in the field of space plasma physics modelling, the results from the MRes project will provide an important demonstration of the differences between stochastic and deterministic modelling and offer ideas of how to shape space weather models moving forward.
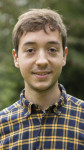
Manuel Santos
Based at: University of Reading
Supervisors: Valerio Lucarini (lead, U. Reading), Jochen Broecker (U. Reading), Tobias Kuna (U. Reading)
Climate is a complex, forced, non-equilibrium dissipative system that can be understood as a high-dimensional dynamical system. Moreover, climate is subject to different kinds of forcing that create fluctuations in the governing dynamics. In our project we shall delve into the so called transfer operator methods in dynamical systems. The transfer operator is a mathematical device that describes the evolution of distributions in phase-space. As such, it captures the information related to the statistics of our system and allows to construct a response theory based on it. I my project, we will concerned with the validity of these methods in a geophysics context. We will study the properties of these operator in coarse-grained phase-space and how they capture information about the (perturbed) dynamics.
By working on phase-space on can construct matrix-approximates of te transfer operator. In particular, we will study the validity of response formulas based on these approximates to investigate its applicability. What is the suitable mathematical framework for these formulas to be valid? How well do they capture the effects of perturbations? Further, since real-world systems are high-dimensional, we will asses the problem of the dimensionality reduction. When the dynamics are projected onto the variables of interest, some of the properties of the transfer operator are lost. What are the mechanisms that provoke the loss of these properties? An answer to these questions will give evidence of the applicability of transfer operator methods in the study of climate putting an emphasis on its structural statistical properties.
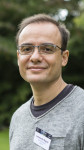
Leonardo Ripoli
Based at: University of Reading
Supervisor: Valerio Lucarini (Department of Mathematics and Statistics, University of Reading)
Co-advisor: Paul Williams (Department of Meteorology, University of Reading), Niklas Boers (Grantham Institute - Climate Change and the Environment, Imperial College London)
Description: The construction of parameterisation for multi-scale systems system is a key research area for GFD, because the dynamics of atmosphere and of the ocean covers a wide range of temporal and spatial scales of motion (Berner et al. 2017). Additionally, the variability of the geophysical fluids is characterized by a spectral continuum, so that it is not possible to define unambiguously a spectral gap separating slow from fast motions. As a result, usual mathematical methods based on homogeneization techniques cannot be readily applied to perform the operation of coarse graining. As shown in recent literature (Chekroun et al. 2015, Wouters and Lucarini 2012, 2013, Demayer and Vannitsem 2017, Vissio and Lucarini 2017), the lack of time scale separation leads unavoidably to the presence of non-markovian terms when constructing the effective equations for the slower modes of variability - which are those we want to explicitly represent - able to surrogate the effect of the faster scales - which are, instead, those we want to parameterise.
Two methods have been proposed to deal effectively and rigorously with this problem:
1) The direct derivation of effective evolution equations for the variables of interest, obtained through a perturbative expansion of the Mori-Zwanzig operator (Wouters & Lucarini 2012, 2013);
2) The reconstruction of the effective evolution equations for the variables of interest though an optimization procedure due to Kondrashov et al. (2015) and Chekroun et al. (2017).
Both methods (which we refer to as top-down and bottom-up, respectively) lead to the definition of parameterisation including a deterministic, a stochastic, and a non-markovian (memory effects) component. The two methods are conceptually analogous, but have never been compared on a specific case study of interest. The MSc project here proposed builds upon the earlier results of Vissio and Lucarini (2017) and deals with constructing and comparing the two parameterisation for the 2-level Lorenz ’96 system, which provides a classic benchmark for testing new theories in GFD. The goal will be to understand merits and limits of both parameterisations and to appreciate their differences in terms of precision, adaptivity, and flexibility.
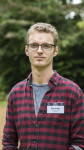
Ben Ashby
Based at: University of Reading
Supervisors:
Tristan Pryer – University of Reading (Lead Supervisor)
Alex Lukyanov – University of Reading
Cassiano Bortolozo – Brazil, Centro Nacioal de Monitoramento e Alertas de Desastres Naturais (CEMADEN)
Summary of the project: Landslides are extreme events that occur when the topsoil on a hill becomes weakened. The result of this can be devastating, both through loss of life and also economic damage. In 2011 a series of floods and mudslides took place in the state of Rio de Janeiro, Brazil. This catastrophe caused over 900 people to lose their lives. This was the driving force behind the creation of the National centre for natural disaster monitoring and alerts (CEMADEN).
For my MRes project, I applied a simple adaptive scheme to numerically solve a simplified PDE model of flow in a porous medium. Data was collected by CEMADEN in an area considered to be at risk from landslides and incorporated into the model to test its sensitivity to the huge variation in soil parameters that determine the flow. Mesh adaptivity was informed by rigorous error estimates involving only the problem data and the numerical solution. Deriving such estimates is known as a posteriori error analysis. The resulting mesh was found to capture the influences of the multiscale data on the solution quite well, but with some undesirable numerical artefacts.
The model used, however, was a heavy simplification. Thus, one of the first steps of my PhD research will be to investigate strategies for the numerical solution of more realistic PDE models with finite element methods. The PDE is degenerate and nonlinear, meaning that even obtaining a numerical solution is much more difficult, and standard techniques for a posteriori analysis cannot be readily applied. If error bounds can be derived, the model will then be tested with mesh adaptivity on data collected during our visit to CEMADEN in Brazil in August 2018. The aim is to create a model to efficiently simulate conditions in the soil so that the team at CEMADEN can use this to inform their work, in which they are responsible for issuing warnings if they believe a landslide is imminent.
As the research progresses, we hope to work more closely with CEMADEN to both use data that they collect and try to adapt our work towards their specific needs in landslide prediction, with the end goal being to provide an accurate and efficient model, informed by the needs of the users.
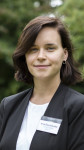
Ieva Dauzickaite
Based at: University of Reading
Supervisors: Peter Jan van Leeuwen (lead supervisor, Department of Meteorology, University of Reading), Jennifer Scott (Department of Mathematics and Statistics, University of Reading), Amos Lawless (Department of Mathematics and Statistics, University of Reading).
Summary of the MRes project: Geophysical systems can be characterised as high-dimensional, nonlinear, with complex feedbacks among a multitude of scales. Understanding the working of these systems, and predicting their future behaviour is a huge challenge. Solid progress has been made through analytical analysis, but computer simulations are an essential ingredient for research and predictions. Unfortunately, these simulations tend to drift quickly and strongly from reality.
Incorporating observational information in these models via data assimilation would allow us to study the true evolution of the system in unprecedented detail, and provide accurate forecasts. Data-assimilation is used routinely for numerical weather forecasting.
The main workhorse is 4DVar, a variational method that tries to find a best trajectory over a certain assimilation window, typically of 6 to 12 hours. The main bottlenecks of this method are the difficulty to make the computations parallel, the inability to make the assimilation windows longer because of the chaotic nature of the atmosphere, and the difficulty in obtaining proper uncertainty estimates.
A solution to the first two problems is to allow for model errors in the data-assimilation framework. This will allow for parallelisation and reduce the strong dependence to initial conditions, making the problem less nonlinear. The last problem can be addressed via an ensemble of 4DVars. A natural way is to treat each 4DVar as a draw from a proposal density in a particle filter.
We propose to investigate efficient solution methods for this minimisation problem, also exploring the fact that similar problems have to be solved in parallel for the different particles in the particle filter. If successful this would not only be a significant step forward in particle filtering, but also lay a solid foundation for the present ensemble methodology used by ECMWF and the Met Office, potentially leading to large improvements in weather forecasting.
This PhD project will provide strong mathematical foundations for this new class of minimisation problems in high-dimensional systems with the aim to make them robust for practical applications. The work will consist of both mathematical explorations, such as convergence proofs and developing the methods further, and numerical experiments on medium to high-dimensional systems, with the aim to eventually reach out to operational data-assimilation practice. For the data-assimilation experiments we will make use of the Parallel Data Assimilation Framework (PDAF) software, developed at AWI and now being incorporated into NCEO plans, which allows different data-assimilation algorithms to be tested on a range of models.
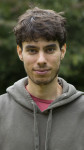
Sebastiano Roncoroni
Based at: University of Reading
Supervisors: Dr. David Ferreira (Lead supervisor) and Dr. Maarten Ambaum (University of Reading, Meteorology), Dr. Valerio Lucarini (University of Reading, Mathematics)
Summary: The Southern Ocean is remote in location, but plays an important role in the global climate system: for example, it absorbs up to 75% of heat and up to 45% of carbon produced by human activity. As observations show that winds blowing over the Southern Ocean (which drive its circulation) have strengthened and shifted poleward in the past few decades, it is natural to ask whether it will continue absorbing heat and CO2 at the same rate. Furthermore, an increase of sea ice cover in the Southern Ocean has been observed during the same period of time, in stark contrast with the decreasing trend observed in the Northern Hemisphere, and this effect is attributed to wind stress modification too. Coupled ocean-atmosphere global circulation models, however, predict that this tendency will invert in the future, but the typical time-scale of the process is still a matter of debate. For these reasons, understanding and constraining the intensity and the time scales of the response of the Southern Ocean is a crucial topic in research.
A wide range of studies have investigated the equilibrium response of the Southern Ocean to wind changes, revealing that its sensitivity is significantly damped by interactions between mesoscale eddies (i.e. turbulent motion) and mean flow. However, a few recent works have also shown that the response actually comprises a superposition of multiple timescales, ranging from one month to more than a decade. Therefore, to capture past and future decadal trends it is essential to consider the transient adjustment of the Southern Ocean, and not just its equilibrium response.
The aim of my project is to discuss the physical processes and time scales involved in the transient response rigorously. From a physical perspective, the eddy-mean flow interaction may be described as a nonlinear oscillatory dynamical system which has already been successfully employed to study storm track variability. All existing models of the Southern Ocean response are linear, but it is vital to explore the nonlinear regime too. This will be used to complement and guide the interpretation of numerical step-change experiments conducted with a high resolution global circulation model. Finally, response theory and nonequilibrium statistical mechanics are powerful tools to investigate the response of a complex climate system to modifications of a forcing parameter, and I plan to extend this approach to the dynamics of the Southern Ocean
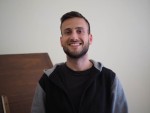
Marco Cucchi
Based at: University of Reading
MPE CDT Aligned student
Supervisors: Valerio Lucarini (lead supervisor) and Tobias Kuna
Project Abstract: In this project I’m going to investigate extreme events in simplified atmospheric models of the mid-latitudes using the point of view of Extreme Value Theory (EVT; Coles 2001). The idea here is to extend the work Felici et al. (2007a, 2007b), where it was first shown that EVT can be used to look at extremes generated by an atmospheric model, going beyond the diagnostic analysis, and taking advantage of the theoretical framework presented in Lucarini et al. (2016). I’m going to investigate the properties of extremes of observables where different levels of spatial and temporal coarse graining procedures are performed, so to understand the effect of averaging on our estimates of extremes. Additionally, statistics of extremes of coarse grained fields will be compared with what obtained running models with coarser resolution. Finally, I will investigate the response of the extremes to both time-independent and dependent perturbations affecting the dynamics, using response theory and pullback attractors. Throughout this work both deterministic and stochastic perturbations will be investigated, and results will be used for model error assessment and analysis of multiscale effects.
As a practical application, this work will lead to the definition of functions describing societal and economic impact of extreme climatic events, along with financial and insurance tools able to manage time-dependent risk assessment.
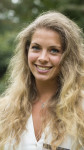
Jennifer Israelsson
Based at: University of Reading
Supervisors: Prof. Emily Black (Lead supervisor, Department of Meteorology, University of Reading), Dr. Claudia Neves (Department of Mathematics and Statistics, University of Reading)
Farmers in Africa are highly vulnerable to variability in the weather. Robust and timely information on risk can enable farmers to take action to improve yield. Ultimately, access to effective early warning improves global food security. Such information also forms the basis of financial instruments, such as drought insurance. Monitoring weather conditions is, however, difficult in Africa because of the heteorogeneity of the climate, and the sparcity of the ground-observing network. Remotely sensed data (for example satellite-based rainfall estimates) are an alternative to ground observations – but only if the algorithms have skill across the whole rainfall distribution; and if the rainfall estimates are integrated into effective decision support frameworks. Current satellite-based rainfall works well for estimating occurrence and low and medium intensity rainfall, but has low rarely estimate heavy rainfall.
Rainfall is often assumed to come from a gamma distribution, which fits well to the low and mid intensity rainfall, but underestimates the probability of heavy rainfall. To more accurately model the tails, we apply the method of “Extreme value statistics”, using both the “Block maxima” and “Peak-over-threshold” method.With this method, only the largest values in the data are used, which makes it suitable for modelling changes in the most adverse events due to, for example, climate change.
In this project we will assess the effect of climate change on the likelihood of extreme rainfall/temperature events in Africa, and subsequently of adverse agricultural outcomes. We will do so by modelling the probability distributions of gauge observations TAMSAT V3 and reanalysis data, with a focus on return periods for extreme rainfall and assessment of uncertainties in return periods for extreme rainfall.This analysis will also be extended to modelled datasets for the historical period, including the new ultra-high resolution (~4km horizontal resolution) CP4Africa and other high resolution data as well as CMIP5. In addition to evaluating the behaviour and representation of heavy rainfall in these dataset, bivariate extreme analysis of temperature and precipitation will be conducted to evaluate the effect of a warming climate on precipitation. The results will be applied to TAMSAT calibration algorithms and to improve climatologies for the TAMSAT-ALERT risk assessments.
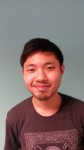
So Takao
Based at: Imperial College London
MPE CDT Aligned student
Supervisor: Darryl Holm
Research Interests: Point Vortex Dynamics, Turbulence, Geophysical Fluid Dynamics, Stochastic Analysis, Symmetry and Reduction in Hamiltonian and Lagrangian Systems, Differential Geometry
Research Project: My current research ideas lie at the intersection of 2D point vortex dynamics and geometric mechanics. Firstly, my idea is to explore a stochastic theory of the motion of point vortices based on the recent work of Holm (2015) on deriving stochastic fluid models using techniques from geometric mechanics, in order to help understand the phenomena of vortex crystal relaxation in 2D turbulence of inviscid fluids. Vortex crystal formation has been observed repeatedly in experiments on magnetized electron columns, which is governed by the same equations as ideal fluids, and in numerical simulations of point vortices, but their formation process is not completely understood. Modelling the weak background vortices as noise may help us give insight to this formation process. Secondly, I am thinking about controlling the motion of point vortices on a curved surface (sphere, paraboloid, etc) by rigid body motion. This can be seen as a generalisation of for instance the motion of point vortices on a rotating sphere.
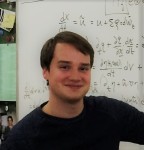
Erwin Luesink
Based at: Imperial College London
Supervisors: Darryl Holm (Lead Supervisor, Department of Mathematics, Imperial College London), Dan Crisan (Department of Mathematics, Imperial College London), Colin Cotter (Department of Mathematics, Imperial College London)
Summary: Weather and ocean prediction requires solving the equations of fluid dynamics. However, our incomplete understanding of turbulence and other subgridscale effects, the chaotic nature of these equations as well as the changing climate are several factors that make solving these equations incredibly difficult. By means of introducing stochastic transport noise [Holm2015] in the equations of geophysical fluid dynamics we will try to improve weather forecasting, but more importantly also provide a proper estimate of the uncertainty in the forecasts.
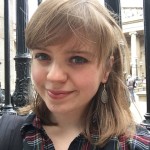
Mary O’Donnell
Based at: Imperial College London
MPE CDT Aligned student
Research project: Vortices are near-ubiquitous geophysical and astrophysical phenomena. The study of vortices which occur in Earth’s oceans is crucial to our understanding of oceanic currents and climate, in part because the majority of kinetic energy of the ocean is contained within mesoscale vortices, but our fluid dynamical understanding of them is constrained both experimentally and practically. This research project aims to model turbulence in quasigeostrophy and describe, kinetically and statistically, the vortex population which naturally arises. An understanding of vortices modelled in this way should provide insight into the population of vortices which arises due to similar flow regimes in the ocean.
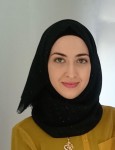
Maha Hussein Kaouri
Based at: University of Reading
MPE CDT Aligned student
Research project: My research will focus on variational data assimilation schemes where we aim to approximately minimize a function of the residuals of a nonlinear least-squares problem by using newly developed, advanced numerical optimization methods. As the function usually depends on millions of variables, solving such problems can be time consuming and computationally expensive. A possible application of the method would be to estimate the initial conditions for a weather forecast. Weather forecasting has a short time window (the forecast will no longer be useful after the weather event occurs) and so it is important to choose a method which gives the most optimal solution in the given time. This is why the analysis of the efficiency of new techniques is of interest. In summary, the aim of my PhD research is to apply the latest mathematical advances in optimization in order to improve the forecast made by environmental models whilst keeping computational cost and calculation time to a minimum.
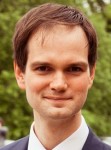
Philip Maybank
Based at: University of Reading
MPE CDT Aligned student
Research project: In Neuroscience, mean-field models are nonlinear dynamical systems that are used to describe the evolution of mean neural population activity, within a given brain region such as the cortex. Mean-field models typically contain 10-100 unknown parameters, and receive high-dimensional noisy input from other brain regions. The goal of my PhD is to develop statistical methodology for inferring mechanistic parameters in this type of differential equation model.
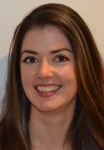
Karina McCusker
Based at: University of Reading
MPE CDT Aligned student
Research project: Fast, approximate methods for electromagnetic wave scattering by complex ice crystals and snowflakes. The goal of my PhD is to develop a method to approximate the scattering properties of ice particles in clouds. This could be used to improve scattering models that are available, and therefore allow more precise retrievals of ice cloud properties. These retrievals could be used to evaluate model-simulated clouds and identify problems that exist in the model, thus enabling improvements to be made to the parameterization of ice processes.
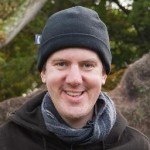
James Shaw
Based at: University of Reading
MPE CDT Aligned student
Research project: Next-generation atmospheric models are designed to be more flexible than previous models, so that the choice of mesh and choices of numerical schemes can be deferred or changed during operation (Ford et al., 2013; Theurich et al., 2015). My PhD project seeks to make numerical weather and climate predictions more accurate by developing new meshes and numerical schemes that are suitable for next-generation models. In particular, the project addresses the modelling of orographic flows on arbitrary meshes, focusing on three aspects: first, how orography is best represented by a mesh; second, how to accurately advect quantities over orography and, third, how to avoid unphysical solutions in the vertical balance between pressure and temperature.
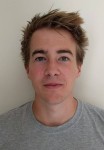
William Mcintyre
Based at: University of Reading
MPE CDT Aligned student
Research project: Atmospheric convection occurs on length scales far smaller than the grid scales of numerical weather prediction and climate models. However, as the resolution of modern models continues to increase, the local convective effects become evermore significant and, thus, there is a demand for new convection schemes which can produce accurate results at these new scales. One such candidate is through conditional averaging, an approach in which grid boxes are split into convectively stable and unstable regions where separate differential equations are solved for each. The scheme incorporates mass transport by convection and memory which are features often ignored in current models. There is thus a possibility to better represent convection using this new approach.
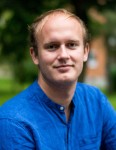