MPE CDT student Mariana Clare has recently finished her internship at LOCEAN CNRS lab in Paris where she was working alongside Redouane Lguensat (LOCEAN), Julie Deshayes (LOCEAN), Maike Sonnewald (Princeton) and V. Balaji (Princeton). She was also the five winners for 2021 for high-level visiting research fellowship!
The original press release can be found here.
Below is the project Mariana was working on:
Should I trust my neural network?
Assessing uncertainty in neural network predictions of ocean circulation under global heating.
In the coming decades, global heating will undoubtedly lead to changes in ocean circulation
potentially pushing the climate beyond key tipping points. However, how ocean circulation patterns
will change is still unclear and there is also a need for novel techniques which can deal with the
uncertainty associated with climate change prediction. In my project I worked with domain experts
at LOCEAN to use Bayesian Neural Networks (BNNs) to provide a probabilistic prediction of the
ocean regime. This probabilistic prediction provides researchers with a way of assessing how much
they should trust the result. Figure 1 shows the accuracy of my BNN as well as the entropy of the
results. Higher values of entropy indicate that the BNN has greater uncertainty about the prediction
there and therefore that the result should be treated with caution. Notably, comparing the two
panels shows that the highest uncertainty occurs where the BNN predicts the incorrect regime
which is a promising result.
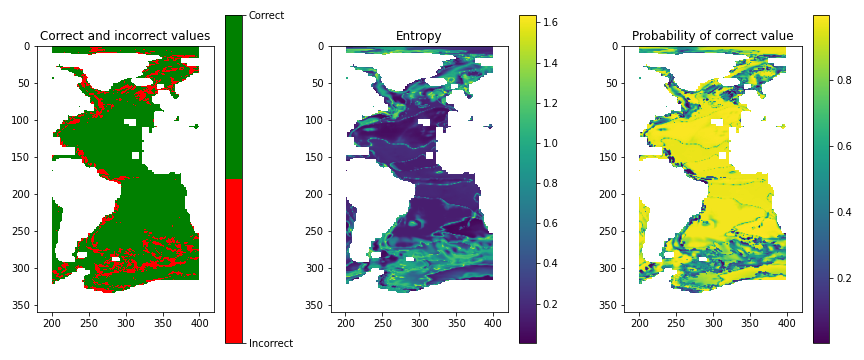
Results gain considerable value to oceanographers and indeed the wider community if their source
and skill is physically interpretable. Techniques to address this issue are known as “explainable AI”,
but there has been very limited research into applying them to BNNs. Therefore, in this project I
explored how conventional methods such as SHAP can be adapted to make them suitable for BNNs.
Figure 2 shows an example of the SHAP values for a specific datapoint. The oceanographers at
LOCEAN were able to confirm that the BNN’s decision making process was physically realistic.
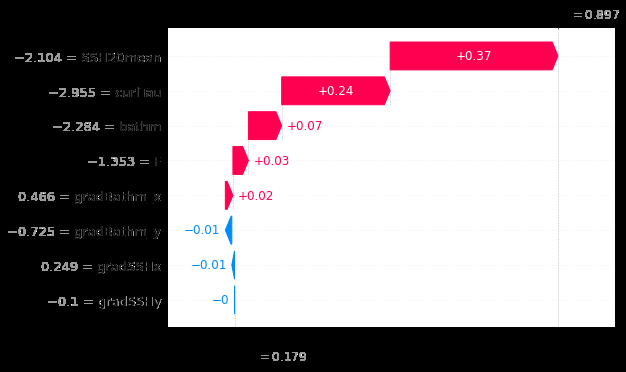