MPE CDT Student Cohort 2014
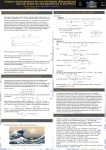
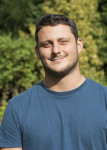
Francesco Ferrulli
Based at: Imperial College London
Research project: Analysis of perturbations for non self-adjoint partial differential operators. Spectral properties and applications to the study of the RSW equation
Supervisors:
Ari Laptev (Imperial College London, Department of Mathematics)
Michael Levitin (University of Reading, Department of Mathematics and Statistics)
In the formulation of the two dimensional Shallow Water equation within a Rotating frame (RSW) the geometry of the problem appears to be substantially changed compared to the same problem settled in the irrotational case. As recognized in the literature, the rotating term acts as a breaking up term for the symmetry of the system so that the resulting system consists in a hyperbolic, non symmetric system of equations. From a spectral point of view the broken symmetry gives rise to a non self-adjoint operator for the linearised problem whose spectrum contains now a purely complex component, which in turn generates in the time dependent context phenomena such as blow-up or formation of turbulences. It is well known that the latter is a feature which appears in many different weather and climate related scenarios. Turbulences are indeed essential to study the cyclone's pattern formation as well as in the context of unpredictability and chaotic systems with particular relevance to numerical simulations. This wide collection of problems arising in different contexts can be allocated under the same very challenging mathematical problem, namely the study of resonances for operators which in case of complex perturbations turns out to be genuinely related to the eigenvalues of a non self-adjoint operator. Recently, new results for two-dimensional complex non self-adjoint Dirac like operators have been achieved in terms of partial description of the complex part of the spectrum and specifically regarding the arrangement of the complex eigenvalues. This PhD project aims to gain knowledge on the solutions of the RSW equations from the study of the linearised system. In order to do so, I will exploit the mathematical techniques that have been used in the study of the spectral analysis of non self-adjoint operators which present similar features to the one arising in the RSW framework. In particular I will be interested in the characterisation of the spectrum and the study of its stability under small perturbations as well as the formation of resonance effects. On the mathematical side, the latter phenomenon turns out to be very challenging and of high interest as pointed out within the KAM theory since it introduces small divisors in the approximating series for the solution of the linearised system, increasing hugely the difficulty of deriving estimates for the series. Furthermore, on the physical side, the linearised shallow water equation system has been recognised as a valid approximation for modelling the propagation of a tsunami wave far from the shore. So the study of resonances of the operator in the linear case itself represents again an interesting topic which can lead to improve the knowledge of the behaviour of such type of waves.
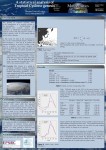
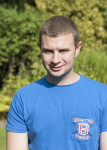
Thomas Leahy
Based at: Imperial College London
Research project: Stochastic and Statistical Modelling of Extreme Meteorological Events: Tropical Cyclones
Supervisors:
Axel Gandy (Imperial College London, Department of Mathematics)
Ralf Toumi (Imperial College London, Department of Physics)
Catastrophe models are essential tools for insurance and reinsurance companies. These models provide support to (re)-insurers calculating an adequate level of capital to cover an extreme meteoro-logical event, a seismic event etc. Catastrophe models are invaluable tools in actuarial science when conducting risk analysis for insurance purposes and are crucial to the managing catastrophe risk expo-sure. The output from these models is used by insurers and re-insurers to make premium and reserve calculations. The reserves set aside are imperative for the reconstruction of housing and infrastructure damaged and destroyed by such extreme events. Due to the power and sophisticated nature of these models, they are very expensive for insurers to lease. One of the inputs to such models is the historical observations of the event, for example historical tropical cyclones. However, since we only have one observable earth, we only have a finite set of reliable observations. This directly influences the level of confidence we have on decisions made based on this data. It is therefore necessary to supplement the historical observations and include synthetic data to improve the level of confidence on the output of catastrophe models. There are models in operation that can achieve this task already, namely Global Climate Models (GCMs). However, depending on the temporal and spatial resolution of the GCMs, they are computationally expensive and time consuming to run. There is also no guarantee that a GCM run will produce the desired event.
The primary focus of this project is to develop a stochastic model for the genesis, propagation and termination of tropical cyclones. Stochastic models can be run with basic computing power and can produce significantly more events in a much shorter time scale. Stochastic models are good at replicating summary statistics of historical tropical cyclones [Leahy, 2015], however in order to quantify possible future scenarios, the stochastic model will have to depend on some physical covariates, for example the sea surface temperature. Reanalysis data is readily available on may physical variables through the ECMWF. One of the aims of this project is to predict the genesis (frequency and location) of tropical cyclones in the future.
To analyse the important factors in the genesis of tropical cyclones, exploratory data analysis and computational statistical methods. Geostatistics and spatial point processes will play an important role in the simulation of synthetic genesis locations. For propagation and termination, research into the appropriate statistical techniques is required.
In past literature, the development of stochastic models for the generation of synthetic tropical cyclones has relied upon the assumption that simulated cyclones will have the same behaviour as historical tropical cyclones [Vickery et al., 2000], [Emanuel et al., 2006] and [Hall and Jewson, 2007]. This boot-strapping like method is justified if one requires tropical cyclones with the same behaviour, however if one wishes to determine what will happen in the future, this assumption is clearly inade-quate. In a changing climate with several possible future scenarios, one requires a model that can by flexible enough to account for possible future climatic scenarios.
The initial basin of interest is the South East Asia and the western-North Pacific ocean. An objective of this project is develop a model that can be applied in different basins with the intention of ultimately developing a global model.
For model validation, we will compare the model to the state of the art and observational data.
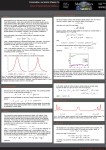
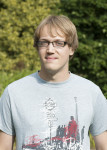
James Jackaman
Based at: University of Reading
Research project: Mimetic discontinuous Galerkin methods
Supervisor:
Tristan Pryer (University of Reading, Department of Mathematics & Statistics)
The goal of this project is to design numerical schemes that preserve certain quantities of interest. A natural question to ask is how many can be embedded in the scheme at once and what effect does this have on the accuracy of the dynamics of the problem.
The three main difficulties in the design of such schemes that will be tackled in this project. They are:
1. Design of appropriate timestepping schemes.
2. Design of appropriate spatial discretisations on planar domains.
3. Extension of these schemes to physically relevant models.
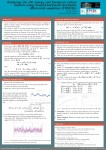
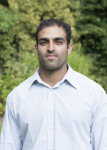
Hinesh Chotai
http://www.imperial.ac.uk/people/hinesh.chotai09Based at: Imperial College London
Research project: Forward-backward stochastic differential equations and applications to carbon emissions markets
Supervisors:
Dan Crisan (Imperial College London, Department of Mathematics),
Mirabelle Muûls (Imperial College London, Business School and Grantham Institute),
Jean-Francois Chassagneux (Université Paris Diderot)
As a response to the risk of Climate Change, carbon markets are currently being implemented in several regions worldwide. Since 2005, the European Union (EU) has had its own Emissions Trading System (ETS), which today is the largest such market. In September 2015, it was announced that China (whose carbon emissions make up approximately one quarter of the global total) will introduce a national emissions trading scheme in 2017. When it comes into effect, China’s market will be the largest of its kind, overtaking the EU ETS. At that point, some 40% of global emissions will be covered by cap-and-trade schemes. According to the World Bank, the world’s emissions trading schemes are currently valued at about $30 billion. However, scientific, and particularly mathematical, studies of these carbon markets are needed in order to expose their advantages and shortcomings, as well as allow their most efficient implementation.
In this project, we will consider a mathematical model for the pricing of emissions permits. The model has particular applicability to the trading of European Union Allowances (EUAs) in the EU ETS but could also be used to model other cap-and-trade schemes. We will investigate mathematical properties such as the existence & uniqueness of solutions for the pricing problem, stability of solutions (e.g. the sensitivity of prices under small perturbations to the market) and their behaviour, as well as computational algorithms for solving pricing problems. The model can be extended to account for multiple trading periods.
The pricing problem that arises from the model fits into the theory of fully coupled forward-backward stochastic differential equations (FBSDEs). The pricing FBSDE is non-standard in several aspects. Firstly, the terminal condition of the backward equation is given by a discontinuous function of the terminal value of the state driven by the forward equation. This is in contrast to the majority of FBSDE literature in which the terminal condition is assumed to be Lipschitz continuous, but appears naturally when considering a market for emissions allowances. Secondly, the forward dynamics may not be strongly elliptic, not even in the neighbourhood of the singularities of the terminal condition.
The project will involve three work packages. Firstly, the project will consider a qualitative analysis of the class of FBSDEs introduced above. As demonstrated in the literature already, this may require one to relax the notion of solution to a FBSDE. Secondly, numerical schemes applicable to the pricing problem will be investigated. The pricing problem is high-dimensional (4-8 dimensions) and a robust numerical scheme will be required to produce satisfactory numerical results, particularly if the multi-period model is to be considered. Thirdly, a case study of the UK energy market will be considered. This will involve calibrating the model’s parameters and processes to real data.
With action on climate change being strongly needed, and the implementation of carbon markets a promising policy option, this research will not only innovate on the theoretical side, but also has a high policy and economic impact potential.
Click on the link below to view Hinesh delivering his pitch at the Imperial’s 2016 3MT competition:
https://www.youtube.com/watch?v=0HHWqI_rQ3I
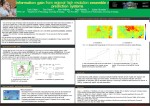
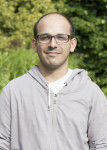
Carlo Cafaro
Based at: University of Reading
Research project: Information Gain that High Resolution Models Bring to Probabilistic Weather Forecasts
Supervisors:
Thomas Frame (University of Reading, Department of Meteorology)
John Methven (University of Reading, Department of Meteorology)
Jochen Broecker (University of Reading, Department of Mathematics & Statistics)
Nigel Roberts (UK Met Office)
Probabilistic forecasting is common in many fields such as financial trading, bookmaking and Numerical Weather Prediction (NWP). Amongst these applications NWP is unique as it is the only one in which the future events in question are governed by physical laws. Probabilistic forecasting is necessary in NWP because: i) the physical laws are chaotic with the result that initial uncertainties grow rapidly with time, and ii) the physical laws are approximated using numerical methods in computer models. Sensitivity to initial conditions means that many different outcomes are possible, given our current state of knowledge, and ensembles of forecasts are used to capture the range of different outcomes. Truncation of spatial and temporal scales in numerical models, essential for implementation, introduces uncertainty associated with “sub-grid scale” motions. Very recently probabilistic forecast generated using numerical models at high 1-2km scale resolutions have started to be produced. Such ensembles can only feasibly be run in local area domains using a coarser resolution global model to provide the necessary lateral boundary conditions. In this set up the information about scales resolved by the high resolution local area model that are below the truncation of the coarser resolution global model is constantly being generated by the high resolution model dynamics as the lateral boundary conditions update. For this information to be times specific and lined to the observed initial state of the atmosphere, requires dynamical processes at the fine scale to be strongly controlled by the larger observationally constrained scales. This project aims to identify the predominant dynamical causes of such information transfer and to quantify the resultant information gain obtained from running high resolution ensemble forecasts.
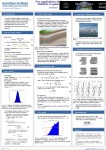
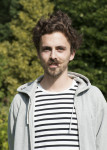
Jens Bendel
Based at: Imperial College London
Research project: Uncertainty quantification in research on green energy
Supervisor:
Ben Calderhead (Imperial College London, Department of Mathematics)
The evidence base for anthropogenic climate change is now unequivocal, and efforts in a multitude of fields are needed to address this urgent challenge. Researchers investigating technologies such as CO2 capture and sequestration, wind power farms or biofuel produced from algae all have to deal with the problem of uncertainty quantification which is inherent to applied sciences.
The large-scale, near-term deployment of CO2 capture and sequestration (CCS) technology is crucial when the production of CO2 cannot be avoided. One of the key challenges associated with this technology is its cost – this will need to be appreciably reduced in order to make it more attractive to the traditionally low-margin power generation industry. In the area of process engineering, reliable data describing thermophysical properties of materials are of paramount importance in every aspect of process design, operation and control, both in academia and industry. To this end, there is a vast activity regarding the measurement, modelling and correlation of these data. There are a number of different measurement techniques in common use, which results in these important data have significant uncertainty.
Wind power farms are a well established source of green energy but still a thriving field of research when it comes to the efficiency regarding their design and installation. To determine possible locations for the installation of wind farms as well as for the installation itself borehole measurements are used to investigate the soil on which windmills are built. Conclusions regarding the condition of the soil in between these boreholes come with significant uncertainty.
The aim of this project is to extract useful information from these noisy or intermittent data sets that can be used to dramatically improve efficiency and reduce the overall cost of deployment of these technologies.
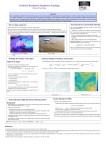
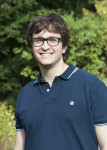
Francesc Pons Llopis
Based at: Imperial College London
Research project: Particle Filtering for applications in Data Assimilation
Supervisors:
Nikolas Kantas (Imperial College London, Department of Mathematics),
Helen Brindley (Imperial College London, Department of Physics),
Dan Crisan (Imperial College London, Department of Mathematics)
Modelling climate dynamics such as ocean circulation or wind turbulence are very difficult research questions with large economic and societal impacts. Quantifying uncertainty in climate prediction and estimating the potential strength of extreme meteorological events is of great importance, even more in the face of global warming. The objective is to provide estimates for spatial and temporal varying phenomena based on a sequence of observations. This task is commonly referred to as Data Assimilation and is of great importance for numerical weather prediction. So far most methods used for this task are based on heuristics, and although they can be tuned to provide fairly accurate estimates it has been not possible to provide any characterisation of the underlying uncertainty of these estimates. Within the scientific communities working in Probability and Statistics, problems like Data Assimilation are usually formulated as high dimensional non-linear filtering problems. Thereby, one aims to compute the probability distribution of a latent Markov process conditional on the collected observations. These so called filtering distributions can provide detailed information about the uncertainty of estimates of the latent process, but are typically intractable and one needs to rely on numerical approximations.
The current state of the art of numerical methods are particle filters also known as Sequential Monte Carlo, which are principled simulation based methods based on successive selection and sampling steps. These are principled methods as they are based on solid theoretical principles, are provably consistent and one could achieve an arbitrary degree of accuracy with enough computational effort. Particle filtering has been extremely successful in many application domains in engineering, economics, systems biology etc., but its success for problems related to Data Assimilation has been rather limited due to the curse of dimensionality. As a result, standard particle methods require an enormous or un-realistic amount of computation to achieve reasonable results. This project aims to assess the potential applicability of particle approximations to challenging problems in Data Assimilation. Early results for filtering problems with the Navier-Stokes equations indicate this is possible when using more sophisticated versions of particle filters that include tempering or carefully crafted Markov Chain Monte Carlo steps.
The aim is to develop advanced particle filters, which also utilise efficiently information from the observations and the model structure. In addition, emphasis will be given to using highly parallelised implementations on appropriate hardware platforms such as Graphics Processor Units (GPU) and computing clusters, which could provide massive computational speed-ups. For the modelling, there are many choices available and one could include various spatial and temporal varying processes from Geophysical Fluid Dynamics. The data sets containing observations of these phenomena can be either synthesised from more sophisticated models or real observations provided by weather forecasting centres.
The methodology developed in the project will be applied to tracking dust storms in the Saharan dessert. Saharan dust storms have a strong impact on the Earth's energy budget. Infrared imagery can be used to highlight their presence and track their movement through space and time. However, the methods used thus far are either subjective, relying on an expert observer, or rather simplistic, using fixed thresholds and taking no account of the temporal element of storm behaviour. In this project the aim will be to develop an analytical approach to identifying and tracking dust storms as identified by the Spinning Enhanced Visible and InfraRed Imager (SEVIRI). If successful it is envisaged that the method will be used to pinpoint dust source locations and their frequency of activation.
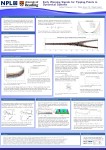
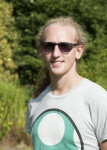
Joshua Prettyman
Based at: University of Reading
Research project: Tipping point analysis of geophysical variables
Supervisors:
Tobias Kuna (University of Reading, Department of Mathematics and Statistics)
Valerie Livina (NPL)
Understanding components of a dynamical system, such as fluctuations and natural/anthropogenic changes in the Earth challenging scientific task. The scale of geophysical monitoring required, the changes due to the development of instrumentation, and the most appropriate analysis methods are the current task under debate. The challenges in studying such time series include being able to handle a large dynamic range, non-Gaussian distributions at various frequency bands, the presence of high-amplitude transients, and processing of large data sets. The uncertainties associated with the trend estimates must account for the nature of the fluctuations as well as the choice of variables, the model, or metrics. In order to understand natural- and anthropogenic-driven climate change, we plan to study Essential Climate Variables (ECVs), in particular, land surface humidity and sea-surface temperature (SST). The goals of this project are to 1) develop a methodology for studying gradual and abrupt changes in geophysical data, by testing and refining previously developed techniques 2) compare these techniques with classical parametric inference approaches for stochastic processes and evaluate the model error by considering higher dimensional models; and 3) determine whether such abrupt changes in the variables under consideration are underway.
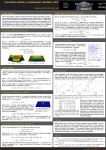
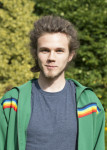
Tobias Schwedes
Based at: Imperial College London
Research project: Uncertainty quantification in systems of partial differential equations with an application in tidal turbine array layouts
Supervisors:
Ben Calderhead (Imperial College London, Department of Mathematics)
Matthew Piggott (Imperial College London, Department of Earth Science & Engineering)
David Ham (Imperial College London, Department of Mathematics)
Simon Funke (Simula Research Laboratory, Oslo)
The regularity of the tides makes them an attractive prospect for clean and secure renewable power production. At multiple locations around the UK the local acceleration of tidal currents makes them potentially suitable sites for the installation of large arrays of tidal turbines. However, these arrays will only be developed if they can be shown to be economically viable. Optimisation of the array size, location and its precise configuration is essential to achieve this, and sophisticated numerical models are required to assist developers. Work in this area to date has focussed primarily on the solution of the shallow water equations, and relatively simple optimisation algorithms. This PhD project will seek to extend the state-of-the-art in optimal turbine array design by using advanced gradient-based optimisation methods, with key novelties coming from the presence of three-dimensional (turbulent) dynamics, the rigorous treatment of system uncertainties, and subgrid-scale turbulent closures.
Three-dimensional effects will require the use of scalable, efficient solution procedures for the Navier-Stokes equations, and here will be based upon code generation techniques developed at Imperial and which are designed to take advantage of the high aspect ratio of our target application to deliver highly efficient discretisation techniques. Part of the project will involve investigation of parameterisation methods to represent tidal turbines (e.g. as momentum sinks, and turbulent sources) within the model. Further to this, both RANS and LES based turbulence closures can and will be considered. Given the scales of the problem and the fact that an inverse energy cascade applies to the depth-averaged dynamics, the use of LES techniques in the horizontal supplemented with RANS models for fully-three-dimensional turbulent dynamics (e.g. providing back-scattering effects) is an attractive and viable large-scale approach that could be considered. The adjoint capability which is key to the optimisation component of this work can also be used to help calibrate these turbulence models to best represent turbine wakes, and thus accurately represent the interactions between turbines within an array. It is clearly crucial to get this right when attempting to optimise the relative locations of turbines.
Uncertainties in the system include imperfect knowledge of bathymetries, the impact of atmospheric conditions of tidal currents, background turbulence characteristics in the water column, and potentially over longer time scales the impact of turbine degradation on array performance. A range of mathematical techniques to deal with these uncertainties will be considered, including Monte-Carlo based or sampling methods, adjoint sensitivity based methods, and both non-intrusive and intrusive versions of polynomial chaos expansions. The latter requires the construction of new solvers for different equation sets governing the coefficients of the polynomial chaos expansion. Historically the time taken to develop new numerical models has been an impediment to this approach. However, here the use of code generation techniques to rapidly develop new models will be considered.
The emphasis of this project will lie on the part on uncertainty.
Click on the link below to view Tobias delivering his pitch at the Imperial’s 2016 3MT competition:
https://www.youtube.com/watch?v=V2xskpw7iDg