MPE CDT Student Cohort 2015
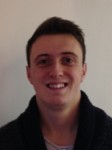
Alastair Gregory
Based at: Imperial College London
Research project: Multilevel ensemble data assimilation
MPE CDT Aligned student
Research project: Multilevel ensemble data assimilation
PhD project summary: Uncertainty appears in a variety of forms in climate and weather models. Many climate and weather models operate on low grid resolutions (which leaves margin for error from sub-grid processes) or have a severe lack of data to guide them to accurate parameter estimates or initial conditions. Ensemble methods aim to incorporate this uncertainty into models to produce high-accuracy estimates by averaging over an ensemble of random (or pseudo-random) model realisations - Monte Carlo estimation. However, to reach the accuracy that most climate and weather models require, an extraordinary amount of realisations of the model have to be computed, and this is very computationally expensive. There is now an alternative: the novel multi-level Monte Carlo method (Giles [2006]). The multi-level Monte Carlo method produces an optimal combination of many low-accuracy samples and few high-accuracy samples to reduce computational cost and variance within the model estimator. Advanced weather and climate ensemble prediction requires high-dimensional spatiotemporal models to be solved accurately, at a low computational cost, and to incorporate a large range of data assimilation techniques - this PhD attempts to achieve this using an adaptation of the multi-level Monte Carlo method.
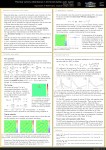
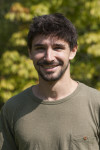
Michael Haigh
Based at: Imperial College London
Research project: Gulf Stream Dynamics in the Shallow Water model
Supervisors:
Pavel Berloff (Imperial College London, Department of Mathematics)
David Ferreira (University of Reading, Department of Mathematics and Statistics)
PhD project summary: This Project is about understanding fluid dynamics of the Gulfstream, which is powerful and important ocean current. The novelty is in terms of extending existing theories from multi-layer quasigeostrophic to multi-layer shallow-water approximation. The challenge is (a) in computing eddy-resolving multi-layer shallow-water solutions of the midlatitude ocean gyres with the western boundary currents and their eastward jet extensions, and (b) in interpreting these solutions with a theory of the nonlinear eddy backscatter. The latter requires mathematical analyses of the ocean circulation responses to transient forcing functions that represent mesoscale eddy stresses.
The mighty Gulf Stream current originates in the Caribbean basin, then follows the eastern coast of the United States up to the Cape Hatteras, where it separates from the coast. After separation, the Gulf Stream continues as the north-eastward jet extension that carries relatively warm water across the North Atlantic towards Europe. The Gulf Stream can be viewed as a giant, ribbon-like ``river in the ocean'' that meanders, sheds enormous vortices called ``rings'', radiates complicated planetary waves and stirs the North Atlantic water masses. Properties of the Gulf Stream flow system are heavily controlled by the Earth rotation and sphericity, by the water density distribution across the ocean, and by the shapes of the coasts and bottom topography. Because of all these factors, dynamical understanding of the Gulf Stream structure, spatio-temporal variability, transport and other properties remains vague, despite more than 50 years of vigorous research on this challenging topic.
The Gulf Stream problem involves important flow interactions across enormous range of scales, from hundreds of meters (internal gravity waves) to thousands of kilometers (ocean basin scale), and from hours to tens of years (meridional overturning and organized decadal variability). Such problem can be most efficiently dealt with by scientific computations guided by the real observations. Nowadays, theoretical research on the Gulf Stream dynamics clusters around 2 main directions. On the one hand, the primitive-equation (i.e., with the minimal number of approximations made) oceanic general circulation models are configured and run in realistic set-ups and eddy-resolving regimes to provide some pioneering solutions. Unfortunately, these solutions are so expensive, that they can not be used for systematic studies of the underlying dynamical processes. On the other hand, theoreticians studying the underlying processes tend to work with overly simplified dynamical models (e.g., the classical quasigeostrophic (QG) model ) that are good for representing some physical processes. Thus, the present situation is extremely beneficial for upgrading the existing theories of the Gulf Stream with more advanced but still fairly idealized models.
This Project will focus on isopycnal (i.e., multi-layer shallow-water) primitive-equation model with just a few layers of constant density --- this is perfect intermediate set-up between ``theoretical'' QG and ``comprehensive'' primitive-equation models. Proposed analyses will be organized into 2 interconnected work programs.
First work program will focus on high-Reynolds-number eddy-resolving solutions of the wind-and-buoyancy-driven ocean gyres, which include the idealized Gulfstream and its eastward jet extension with adjacent recirculation zones. The corresponding solutions will be analyzed from the following angles: flow decomposition into large-scale and eddy components; dynamical balances and nonlinear stresses; statistical description of the flow fluctuations: PDFs, spatio-temporal correlations, Empirical Orthogonal Functions, wavenumber-frequency spectra; nonlinear spectral interactions across scales; energy, enstrophy and potential vorticity analyses: production, propagation, conversion, dissipation; linear-dynamics analysis with local and nonlocal linearizations, stability, impulse responses.
Second work program will focus on fundamentals of the underlying eddy backscatter mechanism that supports the eastward jet and its recirculation zones. The central piece will involve dynamical analysis of the locally forced multi-layer shallow-water solutions represented by linear and nonlinear Green's functions, as well as extensions to various background flows and distributed stochastic forcings.
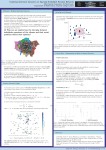
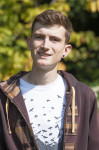
Matthew Garrod
Based at: Imperial College London
Research project: Climate change and climate sentiment
Supervisors:
Nick Jones (Imperial College London, Department of Mathematics)
Robert Ewers (Imperial College London, Department of Life Sciences)
PhD project summary: Public sentiment regarding climate change is an important factor in enhancing and slowing progress towards climate goals. Recent work suggests that in the US, climate change has actually had a beneficial effect with regard to the weather characteristics Americans perceive as important . Climate sentiment itself is a stochastic process running on a social network: large social media studies have observed climate
sentiment but the characteristics of the participants (GPS, socio-economic characteristics) are only partly observed and likewise the social network on which the sentiment dynamics unfolds. The student will investigate the control and forecasting of climate sentiment on spatially embedded networks with partly observed node-locations and unobserved links. Models where individuals are not only influenced by their neighbours but also by the climate field associated with their (partially observed) node-location will be considered. The work will help
create understanding of how climate sentiment unfolds and provide limits on how it can be influenced.
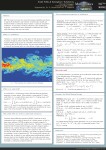
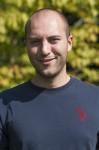
Riccardo Passeggeri
Based at: Imperial College London
Research project: Multivariate ambit fields: Theory, stochastic simulation, statistical inference and meteorological applications
Supervisors:
Almut Veraart (Imperial College London, Department of Mathematics)
Ted Shepherd (University of Reading, Department of Meteorology)
PhD project summary: Multivariate spatio-temporal stochastic processes are of key importance in various applications, including in particular atmospheric and environmental sciences. The aim of this project is to develop the new class of so-called multivariate ambit fields (MAFs) which constitute a flexible, but yet analytically tractable class of multivariate random fields, and derive suitable stochastic simulation and statistical inference tools tailored to applications in the environmental sciences.
The first part of the project consists of deriving the theoretical properties of MAFs, which are defined via kernel smoothing of a multivariate volatility modulated Levy basis; see [BNBV2015, CK2015]. As such MAFs are generally non-Gaussian and allow for stochastic variability both in time and potentially in space. We will in particular study different approaches to parameterising the cross-sectional dependence structure in a parsimonious way.
The second part of the project will focus on developing efficient simulation schemes for MAFs. We will extend methods based on Fast Fourier techniques which are powerful in the univariate set-up, see [NV2016], and quantify the corresponding numerical approximation error.
The third part of the project consists of developing statistical inference tools for MAFs. We will compare composite-, quasi- and simulated likelihood methods in detailed simulation studies.
Finally, we will carry out various data examples: E.g. we will focus on modelling temperature and pressure over a wider region in a bivariate model based on MAFs, see e.g. [GKS2010] for a study of the corresponding forecast errors. Also, we will tailor MAFs to obtain a joint model for solar and wind power production in the European energy market.
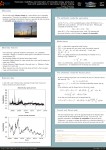
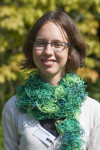
Paulina Rowinska
Based at: Imperial College London
Research project: Stochastic modelling and estimation of renewable energy production data with applications in operational decision making
Supervisors:
Almut Veraart (Imperial College London, Department of Mathematics)
Olivier Feron (EDF R&D OSIRIS, Generation & Market Risk Management Department)
PhD project summary: Weather variables have a huge impact on energy generation based on renewables such as wind and solar which are getting increasingly important in the pursuit of sustainable economic growth. This project aims at constructing stochastic models for renewable energy production data based on appropriate weather variables which are tailored to operational planning and risk management purposes. In addition, efficient methods for statistical inference will be developed which will ensure the applicability of the new models. The scientific contributions of this project will encompass new stochastic models for wind and solar, methodological developments in statistical inference for such models as well as detailed empirical studies.
Climate change threatens the economic prosperity of future generations which makes it urgent to strive for sustainable economic growth, which is in fact one of the key priorities within the UK Sustainable Development Strategy which has been drawn up as a response to Agenda 21 of the United Nations. Mathematics and Statistics play a key role in tackling this challenge and can deliver the reliable tools for risk assessment, which are urgently needed.
The ultimate objective of this PhD project is to develop new technologies in stochastic modelling and statistical inference to reliably quantify risk and uncertainty related to renewable sources of energy production such as wind and solar. While there is a plethora of weather modelling and forecasting methodologies around, see e.g. for a recent survey, such models are typically not tailored to applications in operational decision making which limits their practical appeal in this context.
This project aims to tackle this challenge through a collaborative effort with EDF who will provide expert advice from the perspective of the world-leading power company.
The project will focus on developing statistical models for renewable sources of energy with a particular focus on wind and solar energy production data. Renewable sources of energy are often regarded as notoriously unreliable due to their highly random behaviour which is difficult to predict. However, in order to achieve sustainable economic growth, growing investments in renewable sources of energy are needed and the question big energy suppliers are facing is which investment decision will result in a reliable energy supply for the population while minimising risk at the same time. Given the current situation in Europe, it is particular interesting to find the ideal mix between wind and solar energy production. In order to help with such investment decision, energy providers need to know the corresponding forward prices of electricity and how they depend on the supply and the variations of renewable sources of energy. In this project we will hence focus on deriving suitable stochastic models for wind and solar (and potentially other renewables) which are characterised by two criteria: First, they will need to be flexible enough to accurately describe the random evolution over time of renewables, but at the same time, need to allow for efficient calibration. Second, they will need to be analytically tractable, such that forward prices of electricity generated from renewable sources of energy can be computed in explicit form, which will allow operational decision making based on such models.
Motivated by recent work, see e.g. [3], we will develop suitable continuous-time stochastic process models which can account for non-stationarities in terms of strong seasonal behaviour and trend, stochastic volatility and the existence of jumps. A first starting point will be the class of so-called multivariate volatility modulated Volterra processes, see e.g. [2] for the univariate case. The particular modelling challenge will be to allow for strong (negative) dependencies between wind and solar and also to find a suitable mechanism, which is likely to be via a regime-switching approach, which can model the impact of renewables on the corresponding electricity price.
When it comes to calibration, maximum likelihood estimation will be difficult in the rather complex models mentioned above. Hence we will rather focus on quasi-maximumlikelihood as well as EM-type estimation methods to deal with latent stochastic volatility and stochastic intensities in the underlying jump processes.
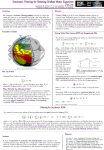
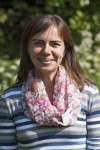
Oana Lang
Based at: Imperial College London
Research project: Data Assimilation for Stochastic Partial Differential Equations
Supervisors:
Dan Crisan (Imperial College London, Department of Mathematics)
Peter Jan van Leeuwen (University of Reading, Department of Meteorology)
Roland Potthast (DWD)
PhD project summary: Climate change is one of the most challenging problems that we are currently facing, fundamental research in this area being, therefore, of crucial importance. Large-scale circulatory motions in oceans and atmosphere involve complex geophysical phenomena that have a determinant influence on climate dynamics. Although it seems impossible to model reality in its entire complexity, the introduction of stochasticity into ideal fluid dynamics appears as a realistic tool for modelling the sub-grid scale processes that cannot otherwise be resolved. We investigate a data assimilation problem for an infinite dimensional model reflecting the motion of an incompressible fluid below a free surface when the vertical length scale is much smaller than the horizontal one. From a mathematical point of view this approach involves a stochastic filtering problem with an infinite dimensional signal modelled by a stochastic partial differential equation, and a large, but finite, observation process. The signal describes the evolution of a rotating two-dimensional shallow water system with the primitive equations. Even if the model is highly simplified in the sense that it does not have the full stratification of the real atmosphere and it involves only a single layer of incompressible fluid, the motions that it supports have close analogues in the real atmosphere and ocean: it allows for processes like gravity and Rossby waves, eddy formation and geophysical turbulence. We will study the influence of missing physics via model noise, while at the same time restricting the evolution by conditioning it on observations of an underlying true system. The observations will be either Eulerian or Lagrangian. The objective of the research is to produce a quantitative and qualitative analysis of the posterior distribution of the state, given the data. The relevance of the results will be investigated by exploring first steps of their realization in an operational framework.
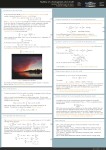
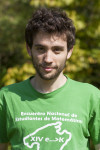
Aythami Bethencourt De Leon
Based at: Imperial College London
Research project: Stochastic GFD: Uncertainty Modelling for Weather & Climate Models
Supervisors:
Darryl Holm (Imperial College London, Department of Mathematics)
Colin Cotter (Imperial College London, Department of Mathematics)
Dan Crisan (Imperial College London Mathematics Department)
Valerio Lucarini (University of Reading, School of Mathematical and Physical Sciences)
Ted Shepherd (University of Reading, Department of Meteorology)
PhD project summary: In next-generation weather and climate models, stochastic parameterisation should be an important element in providing reliable estimates of model uncertainty. A fundamental conclusion of Berner, Jung & Palmer [2012] is that "a posteriori addition of stochasticity to an already tuned model is simply not satisfactory. This in turn suggests that stochasticity must be incorporated at a very basic level within the design of physical process parameterisationsand improvements to the dynamical core." This project will answer the challenge of a priori introduction of stochasticity for GFD models at various levels of approximation, by using the systematic approach of Holm [2015].
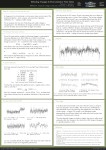
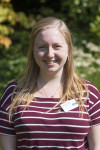
Tasmin Symons
Based at: Imperial College London
Research project: Some functional methods for meteorological time series
Supervisors:
Nick Bingham (Imperial College London, Department of Mathematics)
Nick Klingaman (University of Reading, Department of Meteorology),
Emma McCoy (Imperial College London, Department of Mathematics)
PhD project summary: Recent years have seen the (contested) emergence of a new flavour of El Nino, in the central Pacific. This has led to the suggestion that the atmosphere-ocean coupling in the tropical Pacific has changed: how can we tell? This project will utilise the sophisticated mathematical techniques of Functional Data Analysis to answer
questions about the El Nino Southern Oscillation (ENSO). In this set-up, data points are seen as observations of
a curve. Although this may appear to be a more complicated setting in which to work, we now have access to
powerful mathematical tools, improving our analysis. The aim of this project is to develop this theory further, and apply it to answer questions about ENSO and some related climate processes.
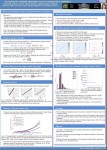
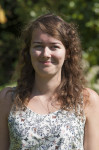
Jemima Tabeart
Based at: University of Reading
Research project: On the treatment of correlated observation errors in data assimilation
Supervisors:
Sarah Dance (University of Reading, Department of Meteorology)
Joanne Waller (University of Reading, Department of Mathematics and Statistics)
Amos Lawless (University of Reading, Department of Mathematics and Statistics)
Nancy Nichols (University of Reading, Department of Mathematics and Statistics)
PhD project summary: Numerical weather forecasts are obtained by evolving forward the current atmospheric state using computational techniques that solve equations describing atmospheric motions and other physical processes. The current atmospheric state is estimated by a mathematical technique known as data assimilation. Data assimilation blends previous forecasts with new atmospheric observations, weighted by their respected uncertainties. The uncertainty in the observations is not well understood, and currently up to 80% of observations are not used in the assimilation because these uncertainties cannot be properly quantified and accounted for. This project will investigate mathematical methods to approximate observation uncertainty that preserve observation information content while being sufficiently efficient for practical use in operational weather prediction.
In Numerical Weather Prediction (NWP), large computational models simulate the complex nonlinear equations of motion of the atmosphere. Forecast accuracy is still constrained by uncertainties in the initial conditions, known as the analysis. Variational data assimilation techniques are often used to compute the analysis by minimizing a nonlinear cost function. This is essentially a weighted measure of the distance from forecast states (the background) and the available observations over a fixed time window, weighted by the uncertainties in the data. Thus for good results, accurate specification of the forecast and observation error distributions is vital.
It is becoming increasingly important to use observation data from remote sensing instruments (e.g., satellites and ground based-radar) providing detailed information about the current state of the atmosphere on fine scales. Although tt is well known that these data have spatially correlated errors, data assimilation algorithms have typically treated the errors as white noise. This approximation is made since the details of the correlation structure are often unknown. It also allows a simplification of the calculations and a reduction in computational cost. Unfortunately, these measures do not fully exploit the observations and significant information may be lost in the assimilation.
More recently, we have shown that it is possible to estimate observation error correlations. A proper treatment of observation error correlations results in more accurate analyses and improvements in forecast skill. However, estimates of observation error correlations are often noisy, and it is unclear the best way to regularize these to ensure speedy convergence of the iterative scheme used to minimize the cost function and preserve the maximum amount of observation information content.
This PhD project will investigate methods of covariance regularization that preserve observation information content. The first stage of the project will be to consider existing covariance regularization schemes applied to some typical noisy estimated observation error covariance matrices and compare their effects. Metrics to consider in the comparison include observation information content, analysis accuracy and minimization convergence speed. This initial work is expected to provide a basis for the development of a new regularization method or variational assimilation pre-conditioning technique. The project lies within numerical linear algebra and optimization and will consist of theoretical work supported by numerical computations in an idealized framework. There will also be the opportunity to work with real observation and model data from the Met Office and gain an understanding of practical operational issues.
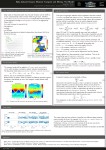
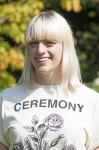
Josephine Park
Based at: Imperial College London
Research project: Material Transport and Stirring in the Ocean
Supervisor:
Pavel Berloff (Imperial College London, Department of Mathematics)
Igor Shevchenko (Imperial College London, Department of Mathematics)
Erik van Sebille (Imperial College London, Grantham Institute/Dept. Physics)
PhD project summary: Ocean currents and eddies constantly transport and stir huge amounts of water masses and their properties. An efficient way of detecting these processes is by releasing neutral floats and tracking
their trajectories. An alternative diagnostics is provided by tracking passive tracer concentrations. On the one hand, the observations show enormous complexity of the eddy-induced transport, which turns out to be not only spatially inhomogeneous and anisotropic but also significantly non-diffusive. On the other hand, general circulation models routinely approximate this transport as homogeneous and isotropic
diffusion. This dire situation ensures great potential not only for upgrading the diffusion approach, but also for developing new, physically consistent and much more accurate, simple models of material transport. The goal of this Project is to investigate material transport and stirring properties in idealized but dynamically consistent and structurally rich eddying-flow simulations, and to use these analyses for developing new generation of simple transport models based on other principles.
The Project will involve simulations of several types of idealized geostrophic turbulence.
Subsequent kinematic analyses of their transport and stirring properties will be used for developing simple stochastic and deterministic models of the transport and stirring for practical applications. The following research agenda is planned:
–- Statistical analyses of ensembles of Lagrangian particles; single- and two-particle dispersion laws,
Lagrangian autocorrelations, Lyapunov exponents, etc.;
–- Estimates of effective diffusivities, quantification of non-diffusive behaviours, transport barriers
and surf zones;
–- Inhomogeneous and anisotropic diffusive modelling of the transport;
–- Direct stochastic modelling of transport (high-order Markov models, Levy flights, fractional Brownian
motion, etc.);
–- Indirect stochastic modelling of transport by random kinematics;
–- Dynamical underpinning for kinematical transport and stirring properties
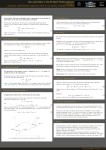
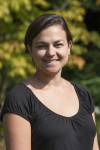
Lea Oljaca
Based at: University of Reading
Research project: Probabilistic and uncertainty information in suboptimal filters applied to dissipative PDE’s
Supervisors:
Jochen Brocker (University of Reading, Department of Mathematics and Statistics)
Tobias Kuna (University of Reading, Department of Mathematics and Statistics)
PhD project summary: The term Data assimilation refers to methods whereby noisy observations of a physical system are incorporated into a dynamical model of that system, for instance a PDE, in order to reconstruct the current state of the system or even entire past orbits. Filters are a subclass of data assimilation algorithms which provide an estimate of the current state based on present and past (but not future) observations. Further, filters are often designed to work recursively, using new observations to update on previous estimates.
Although optimal filters exist in theory, they are essentially infinite dimensional in nature which renders application of these hypothetical algorithms infeasible in practice. A very large number of suboptimal but practically feasible filtering approaches have been proposed, most of them being in one way or another reminiscent of the Kalman Filter, which is the optimal filter in the case of linear systems with Gaussian perturbations. A better understanding of these filters is of utmost importance in all fields of applications such as weather forecasting, but it also leads to interesting mathematical questions related to stochastic dynamical systems and probability theory.
The analysis of filtering algorithms (optimal or suboptimal) essentially evolves around the following three core questions:
1. Is the filter stable in the sense that the internal parameters of the filter stay within the relevant range, or do they diverge to machine infinity in finite time with large probability (see[4])?
2. Is the filter stable in the sense that the initial values of certain parameters such as the initial estimate of the state become irrelevant as time progresses (see[2,3])?
3. What is the accuracy of the filter, either in absolute terms or relative to other algorithms, for instance the optimal filter (see[2,3])?
In the context of geophysically relevant models such as the Navier Stokes equations and its various relatives, all three questions have been studied in the literature.
The accuracy of certain filtering algorithms (Question 3) has also been the subject of the MPECDT MRes project ``Data assimilation in the 2--dim incompressible Navier--Stokes Equation’’, which is a predecessor to this PhD project. That project (and various publications), exploit a remarkable fact shared by many dissipative PDE’s relevant in geophysical fluid dynamics, namely that the projection of the solution onto a suitable finite dimensional space will eventually determine the whole (infinite dimensional) solution.
As far as we can see however, performance analysis has mainly focussed on the filters’ ability to estimate the current state. The potential to provide useful aposteriori error information has often been mentioned and was in fact a major driver behind the development of various filter variants, but there is not much in terms of rigorous analysis of whether this information is in fact reliable. Moreover, it seems that there is no generally accepted methodology whereby such a question could be addressed (see however [1]). The aim of this project is to further develop such a methodology and contributed to filling this gap.
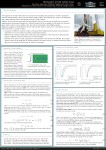
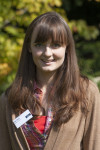
Zoe Goss
Based at: Imperial College London
Research project: Second-generation design tools for the optimal exploitation of tidal renewable energy
Supervisors:
Matthew Piggott (Imperial College London, Earth Science & Engineering)
Colin Cotter (Imperial College London, Department of Mathematics)
Simon Funke (Simula Research Laboratory)
PhD project summary: The regularity of the tides makes them attractive for renewable power production, with the UK at the forefront of a fledgling industry seeking to realise this potential. Advanced numerical tools are required to optimise array designs to maximise power yield and minimise costs and impacts. Substantial progress on the development of such tools has been made in recent years; this project seeks to address some of the outstanding requirements for their real-world application. This includes the implementation of turbulence parameterisation methods, the inclusion of sophisticated financial cost models, and the development of individual turbine control capabilities.
The regularity of the tides makes them very attractive for renewable power production. At multiple locations around the UK the local acceleration of tidal currents provides suitable sites for the installation of large arrays of tidal turbines. However, these arrays will only be developed if they can be shown to be economically viable, feasible from engineering perspectives, and with acceptable environmental impacts. Optimisation of the array size, location and its precise configuration is essential to achieve this (Vennel et. al., 2015), and sophisticated numerical models are required to assist developers in these tasks (Funke et. al., 2014; Culley et. al., 2016).
To date our work in this area has focussed on the use of automatic code-generation techniques (Rathgeber et. al., 2016) for the numerical solution of the depth-averaged shallow water equations and, uniquely, the development of associated adjoint-based optimisation algorithms (Farrell et al., 2013; Funke et. al., 2014). A current PhD project is working towards the inclusion of uncertainty quantification techniques within both power yield estimates as well as ‘robust’ array optimisation algorithms, and another is considering the trade-off between maximising power or profit within an array design and potential environmental impacts.
The focus of this project will be to take the next steps towards the development of a so-called ‘second-generation’ design tool of value to industrial users. In particular, we will address the fact that little effort to date has gone into the parameterisation of subgrid-scale processes, including turbulence. This is of vital importance for reliable estimated of how turbines interact with one another within an array and respond to and alter the ambient environment. This effort will build upon a MRes project considering ‘horizontal large eddy simulations (HLES)’ methods, and extend to three-dimensional RANS and LES methods using the numerical capabilities developed in a parallel ongoing EPSRC project “A new simulation and optimisation platform for marine technology”.
Secondly, we will seek to incorporate improved economic models in order to investigate what impact optimising different economic properties could have on the array, and how these change based upon the priorities of the stakeholder concerned. For example, the public may just want more power generated cheaply but the investors may want to maximise return on investment or prefer a solution that minimises capital cost and therefore risk at the expense of higher generation.
Thirdly, while design of the array is important, there is still a key role for a computational design tool in the optimisation of the ‘operation’ of the array once installed. Here control theory will be utilised to investigate the control of individual turbines (e.g. dynamic control of blade pitch to alter the relationship between flow speed and power production) in order to operate them in such a way that mitigates the effect of nearby device downtime, and potentially minimises the need for maintenance through reducing structural loads. Estimates of, and reductions to, the significant costs associated with device maintenance and downtime in turn feeds back into the second topic.
Each development will be tested on an existing suite of idealised coastal ocean configurations, as well as realistic sites with arrays currently under development.
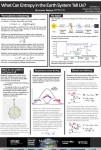
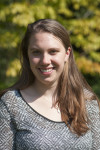
Goodwin Gibbins
Based at: Imperial College London
Research project: Earth’s Entropy Budget
Supervisors:
Joanna Haigh (Imperial College London, Grantham Institute/Dept. Physics)
Valerio Lucarini (University of Reading, Department of Mathematics and Statistics)
PhD project summary: In his landmark book “The Theory of Heat Radiation” Planck (1913) showed that associated with any flux of electromagnetic radiation there is a flux of entropy and, through using an inversion of the black body function for temperature as a function of intensity, the spectrum of radiant entropy can be deduced from the spectrum of radiant energy.
In a climatic steady state the global annual average solar energy absorbed by the Earth is exactly balanced by an equal emission to space of heat energy. It is well established, however, that the entropy flux associated with the emitted radiation is far larger than that of the absorbed solar radiation, with the balance being created by non-equilibrium processes within the climate system.
Different states of the climate are thus reflected in different fluxes of entropy and in this project we investigate the impact on the entropy budget of factors that produce radiative perturbations to the climate, for example changes to the concentration of atmospheric carbon dioxide or to solar irradiance.
To date there has been very little work published on spectral entropy fluxes, and some that has is misleading or of low accuracy. In this project we will use state-of-the-art computer models of atmospheric radiative transfer to calculate the radiation and entropy spectra for various climate change scenarios and thus deduce the entropy production required of the Earth.
We will complement the radiative point of view on entropy production with that based on the analysis of irreversible processes inside the geophysical fluids.
We shall look at the impact of performing spatial and temporal coarse graining in the climatic fields resulting from general circulation models as well as in the spectral resolution of the radiative models. This will inform us on the degree of convergence of the simulated properties with resolution.
We expect to use the output of GCMs available through the PCMDI as well as high resolution runs available in Reading. We also expect to use data resulting from radiative transfer models.
An additional approach will be to calculate the entropy fluxes associated with radiation spectra measured from satellites, or calculated within global climate models.
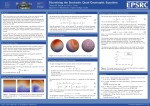
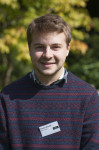
Thomas Bendall
Based at: Imperial College London
Research project: Physics-Dynamics Coupling in Numerical Weather Prediction and Climate Models
Supervisors:
Colin Cotter (Imperial College London, Department of Mathematics)
Ben Shipway (UK Met Office)
Jemma Shipton (Imperial College London, Department of Mathematics)
PhD project summary: In numerical weather forecasts and climate models, the resolved fluid processes are called the 'dynamics', and are separated from the unresolved processes (known as the 'physics'). To reflect the behaviour of the Earth System, these processes communicate to one another through a process called 'coupling'. Currently the UK Met Office is developing a new numerical discretisation for its dynamical core, known as Gung Ho!. This will use a finite element method, which presents a host of issues for the coupling of the new dynamical core with the physics processes. For my project, I will be exploring some of these issues and attempting to find solutions to them.
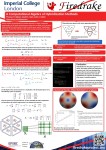
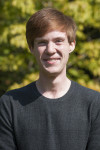
Thomas Gibson
Based at: Imperial College London
Research project: Higher order finite element methods for numerical weather prediction
Supervisors:
David Ham (Imperial College London, Department of Mathematics)
Colin Cotter (Imperial College London, Department of Mathematics)
Thomas Melvin (MET Office)
PhD project summary: Recently developed numerical approximations of the equations of motion for the atmosphere, based on the framework of compatible finite element methods, has been shown to maintain exact force balances which is essential for generating accurate solutions. These approximations allows use to be made of massively parallel supercomputers, and thus are being used in the Gung Ho project to make a new dynamical core for the Met Office and the UK climate science and meteorology community.
This project focuses on the design and analysis of new discretization methods within the compatible finite elements framework, and their implementation in software through the Firedrake project. Current investigations include, but are not limited to:
• Multigrid algorithms for the implicit Helmholtz-type operator for compressible flows, and their analysis and implementation;
• Design analysis of stability and accuracy, and implementation of transport schemes for density, temperature and velocity;
• Development of higher order discretizations and their efficient implementation via sum-factorization or Bernstein polynomial techniques;
• High performance Bernstein embedded DG transport schemes;
• Design, analysis and implementation of efficient solvers for the Helmholtz-type operator in the case of spectral element or Bernstein bases;
• High performance implementation of higher order finite element spaces using automated code generation.